The Human Side of Cybersecurity Seminar Series: John Doyle and Complex Systems
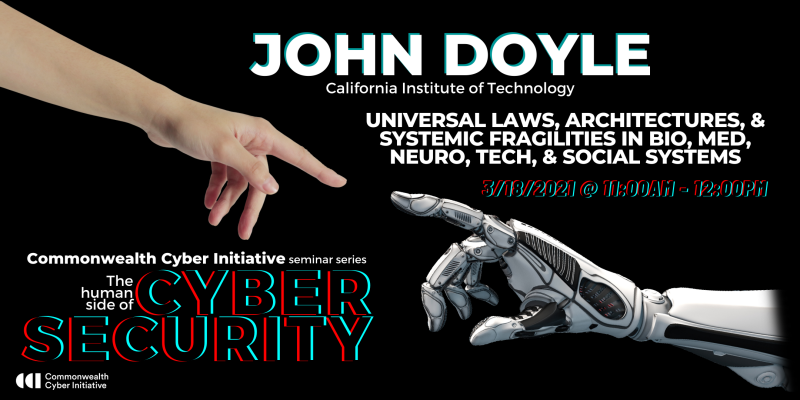
Date:
Thursday, March 18, 2021
11:00 AM – 12:00 PM ET
Caltech’s John Doyle Discusses His Pioneering Work in Complex Systems
Universal Laws, Architectures and Systemic Fragilities in Tech, Bio, Neural, Med, and Social Systems
Presentation Abstract:
The effective layered architectures found in our brains and other organisms seamlessly integrate high-level goal and decision making and planning with fast, lower-level sensing, reflex, and action and facilitate learning, adaptation, augmentation (tools), and teamwork, while maintaining internal homeostasis. This is all despite the severe demands such actions can put on the whole body’s physiology, and despite being implemented in highly energy efficient hardware that has sparse, localized, saturating, delayed, quantized, noisy, distributed (SLSDNQD) sensing, communications, computing, and actuation. Similar layering extends downward into the cellular level, out into ecological and social systems, and many aspects of this convergent evolution will increasingly dominate our most advanced technologies. Simple demos using audience’s brains can highlight universal laws and architectures and their relevance to tech, bio, neuro, med, and social networks.
The past year has unfortunately highlighted the intrinsic and systemic unsustainability and fragility of our social and technological infrastructures. We’ll aim to give novel insights into the mechanisms underlying “systemic fragilities” in our immune, medical, computing, social, legal, energy, and transportation systems, and potential alternative architectures that could be more sustainable and robust.
To do this, we’ll sketch progress on a new unified theory of complex networks that integrates communications, control, and computation with applications to cyberphysical systems as well as neuroscience and biology. Though based on completely different constraints arising from different environments, functions, and hardware, such systems face universal tradeoffs (laws) in dimensions such as efficiency, robustness, security, speed, flexibility, and evolvability. And successful systems share remarkable universals in architecture, including layering, localization, and diversity-enabled sweet spots, to effectively manage these tradeoffs, as well as universal fragilities, particularly to infectious hijacking.
The study and design of systems architectures have traditionally been among the areas of engineering least guided by theory, and there is nothing remotely resembling a “science” of architecture. Our aim is to fundamentally change this with new mathematics and applications, while still gaining valuable insights from both the art and evolution of successful architectures in engineering and biology. Our framework also suggests conjectures about senescence, and tradeoffs in the evolution of cancer, wound healing, degenerative diseases, auto-immunity, parasitism, and social organization, and potential animal models to explore these tradeoffs.
CCI Fellow Milos Manic, director of the Virginia Commonwealth University Cybersecurity Center, will moderate the webinar.
About John Doyle:
John Doyle is the Jean-Lou Chameau Professor of Control and Dynamical Systems, Electrical Engineer, and BioEngineering at Caltech. He received a B.S. and M.S. in electrical engineering, MIT (1977), and Ph.D. in math, UC Berkeley (1984). He was a consultant at Honeywell Systems and Research Center from 1976 to 1990.
Doyle’s research is on mathematical foundations for complex networks with applications in biology, technology, medicine, ecology, neuroscience, and multiscale physics that integrates theory from control, computation, communication, optimization, statistics (e.g. Machine Learning). An emphasis on universal laws and architectures, robustness/efficiency and speed/accuracy tradeoffs, adaptability, and evolvability and large-scale systems with sparse, saturating, delayed, quantized, uncertain sensing, communications, computing, and actuation. Early work was on robustness of feedback control systems with applications to aerospace and process control. His students and research group developed software packages like the Matlab Robust Control Toolbox and the Systems Biology Markup Language (SBML).
His prizes, awards, records, championships include the 1990 IEEE Baker Prize (for all IEEE publications), also listed in the world top 10 “most important" papers in mathematics 1981-1993, IEEE Automatic Control Transactions Award (twice 1998, 1999), 1994 AACC American Control Conference Schuck Award, 2004 ACM Sigcomm Paper Prize and 2016 “test of time” award, and inclusion in Best Writing on Mathematics 2010. Individual awards include 1977 IEEE Power Hickernell, 1983 AACC Eckman, 1984 UC Berkeley Friedman, 1984 IEEE Centennial Outstanding Young Engineer (a one-time award for IEEE 100th anniversary), and 2004 IEEE Control Systems Field Award. Also, he holds world and national records and championships in various sports.
Registration Information
Register here. If you have problems with registration, please contact Susie Kuliasha at susiek20@vt.edu.
Extra Credit Reading: Learn more in advance of John Doyle's presentation
From John Doyle: some notes in preparation for lectures on robust learning and layered architectures for control
These notes are optional background reading for my lecture(s) on the role of humans and automation in cyber-physical systems with an initial emphasis on the cyber end. There are many connections with both old style human factors (e.g. Fitts’ law) and newer issues in cyber-security, sustainable infrastructure, and even social, political, economic, and legal aspects of networks in addition to technology. The start however will be more related to the origins of human factors in WW2 around design of (primarily weapons) technologies with human operators (e.g. pilots, drivers). More generally humans and their interactions with tools and technologies have limitations on system level performance and robustness that can be traced to limitations in their components and architectures. We’ll eventually get to the classic Fitts’ law, but start with some easier and more accessible modern examples.
There are 5 familiar challenges or experiments (and a highly optional 6th) that proximally motivate a new theory of brain architecture and sensorimotor control that is relevant to human factors but also cyberphysical systems (CPS) more generally. Most of the experiments here can be done in just a few minutes, but hopefully you’ll also spend some time trying to explain the results, which I will aim to also do in lectures, and very briefly and superficially below. There is more material below in addition to the experiment descriptions that will also be discussed.
These experiments will also hopefully concretely start motivating a set of related broader ideas around complex network architecture: robustness, fragility, efficiency, robust control, learning, laws and tradeoffs, speed-accuracy tradeoffs (SATs), acute vs chronic, accidental vs systemic, virtualization, layers vs levels, instability and delay, unstable zeros, waterbed effects, diversity enabled sweet spots (DeSS), SLSDNQD (sparse, local, saturating, delayed, noisy, quantized, distributed), system level synthesis (SLS).
If you’ve heard me before on “universal laws and architectures” this written material and the lectures to come will aim to be more accessible and tutorial at the beginning, with some new research directions at the end. Two of the experiments (I won’t ask you to try, but to imagine) are 1) real downhill mountain biking and 2) a video game version we’ve developed as a quantitative experimental platform. We have several papers on this and lots of videos online which I’ll point you to. It isn’t essential that you study these, but they are very illustrative of deeper points connected with our research.
Unfortunately, real and virtual bike experiments require special equipment (and software for game versions) and getting IRB approval to crash mountain bikes would be difficult. Our other 3 experiments (you will hopefully try) are each different simplifications of sensorimotor control in biking, are more qualitative, easy to do at home, totally benign and safe, and have variants you can explore with dramatically different outcomes (e.g. crash or not). Some details:
Mountain biking down a twisting bumpy trail is an illustrative neuro/physiology control problem that humans excel at with intuitively accessible questions and new answers, both theory and experiment. (Search for “extreme mountain biking video” to see some dramatic examples.) In the highest layer primarily in cortex, advanced warning via vision is used to plan and track the trail with small error if speed, terrain, bike, and skill are properly matched. While high layer goals can be conscious, most planning/tracking is still automatic and unconscious. (Note that unconscious and automatic is not the same as model-free, and may in fact often be the opposite, an important point we’ll want to clarify.) The bumps in the trail are handled by a separate reflex layer that is entirely unconscious with unavoidably delayed reactions that must deal with the unstable dynamics of the bumps, the bike, and the rider’s body and include the VOR (vestibular ocular reflex) which stabilizes vision despite bumps and head motion. Both layers have complex communications and computing, and the lower layer also has sensing and actuation, all of which are SLSDNQD (sparse, local, saturating, delayed, noisy, quantized, distributed).
Good athletes learn to multiplex the trail and bump tasks extremely well with modest training (much less than the legendary 10K hours), as it exploits capabilities (including learning and tool use) that humans have evolved in order to become top predators. Our layered architecture enables goals/plans using highly virtualized and stable, predictable models despite the lower layer physics being wildly unstable and unpredictable. Good reflexive bump control makes goals/plans/tracking easier and vice versa. With enough advanced warning, proper equipment, and skill matched to speed and terrain, we can be almost perfectly robust, but with anything less, we can be catastrophically fragile.
With the PC game version, we can adjust the difficult of the trail and bumps and add delays and noise in sensing and actuation, all in software and much more easily than in real biking. Subjects learn with experience to reduce the errors, and while there are large individual differences, everyone (virtually) crashes with sufficiently difficult trails and bumps. (Adding texting, however, would be catastrophic even in easy cases as we are not evolved to multiplex this, and our theory predicts this as well.)
We can also model all these features plus the limits of the human sensorimotor system in terms of quantization, delay, and most importantly, layers (plan vs reflex) and levels (system vs nerve). All the many predictions of the theory are strikingly consistent with experiments, and this is our simplest quantitative match between theory and experiment. Basically, we can systematically adjust the game difficulty in many dimensions, with predictable increases in errors which eventually get large enough to crash if it were a real bike. We have available several papers and videos on the details which you are encouraged to study but will not be essential. Our focus here will be on simpler experiments that you can do at home with minimal equipment but make the same qualitative points about properties of control problems that make them intrinsically hard.
Humans learn much of bike riding in a what appears to be a model-free way, and have difficulty explaining exactly how the trail and bump layers work and interact. We will argue this is one of many illusions we have about our brains and behavior, and the evidence is overwhelming that we have massive, complex internal models of ourselves and our environments. Obvious evidence comes from the complexity of our dreams where we generate rich sensations of complex environments with little or no external sensory inputs. The idea that “seeing is dreaming” is that these same internal models we “see” in dreams are the same models we perceive when awake and seeing. But when awake our visual system generates errors when sensed images and models disagree sufficiently, so the “dreams” are roughly synced to incoming sensation. The architecture of this “model-based” vision and control in humans will be an optional subject branch, and our theory explains much of what is known about internal models and feedback in vision.
While bike riding may create the illusion of model-free learning, if there is a serious accident or crash that is unexpected, we will normally switch to model-based analysis and design. This is a simple example of “accident analysis,” and there are subtleties about what this entails that we may want to explore, but we’ll start simple. A crash or accident could be due to too tight turns, too steep trails, too large bumps, flat tires, broken chains, physical injury, etc., and can produce additional damage. Diagnosing which of these is responsible requires a model-based understanding of their mechanisms beyond what is needed to simply ride. It is analogous to shifting from being a rider/driver to a mechanic or engineer. While high layer symbolic reasoning would be essential to automate such analysis, so would layer-specific models of mechanisms in the reflex and bike layers, and a framework that allows for distinguishing them.
As another example, consider what is involved in “fatigue.” Concretely, suppose you are a competitive runner in a marathon, and you experience an unexpected level of pain, which is a highly virtualized summary signal about the state of lower layers of your physiology. If it is near the end, you might ignore this and speed up, whereas if the same pain was experienced outside of exercise, say, on waking up in the morning, you might plausibly conclude you were ill and seek medical attention. If it is in the middle you might slow down and try to evaluate the source of pain, switching from “driver to mechanic.” So, the pain of fatigue as a highly virtualized summary statistic of lower layer body states illustrates how a kind of layered robust control has evolved in us to avoid physical damage during intense activity. Part of both athletic and medical training is to learn to better interpret pain signals to better balance further damage with maintaining performance. This kind of integration of learning and layering is likely to be essential to create robust learning for control.
The unconscious/automatic reflex layers effectively virtualize all the lower layer hardware. While this is essential to manage this complexity, it can make it difficult to diagnose failures or other changes and learn new controllers to deal with them. So, layering and virtualization can facilitate learning by providing lower layer reflexes that provide stability and robustness to bumps and similar uncertainties, and can simplify the result dynamics that higher layers see, but can also hinder learning by making lower layer dynamics and failures difficult to diagnose and change. Feedback control can both reduce and amplify plant uncertainty, and this will be an essential issue in understanding the limits of learning and control. All of these are important tradeoffs but formalizing them is challenging.
We have developed a simple safe version of mountain biking using standard video gaming hardware and software. This is not only safer and cheaper than reality, but allows manipulation of virtual trails, bumps, bike dynamics, and the interface with the player to create a much richer and more quantitative experiment than could be done in the field. Unfortunately, neither real nor virtual biking is easy for you to try out under the current circumstances. (Though the total biking video game can be quickly and easily built with a laptop and $300 of hardware, and free downloadable software. If anyone is interested, let me know and you can probably borrow one copy of the hardware, which is just a standard video game steering wheel.) I will discuss the results so far, but you won’t be able to try the experiments yourself.
What I’ll focus on here is the other 3 experiments which you will hopefully do at home and will let us explore most of the issues from the biking game in more detail. The first and most complex is upright balance of your body, which involves roughly 3 layers of control: vision, vestibular reflex, and proprioception (Vis, Vest, Prop), each implemented in separate layers of the nervous system. As a child, we must learn to balance and walk and run upright and bipedally. Then later we might learn to additionally balance and steer a bike on simple level terrain. Some then go on to learn to ride mountain bikes and deal with trails and bumps. Here we’ll focus on the simplest problem of balancing.
To make balancing challenging we’ll do it while standing on one foot, which degrades the Prop control, though most people can do this essentially indefinitely. It is then easy to additionally remove Vis by closing both eyes, after which most people eventually fall or open their eyes. (It is easy to stand on two feet with eyes closed, but hard to move without risking collisions.) The time to falling can be quite variable between people and it is possible to improve some with practice. The dependence of time to fall has been studied and shown to roughly correlate with expected remaining lifespan predicted from age and other measures, and can depend on age any existing conditions, as well on fitness, training, natural ability, etc. Hopefully you’ll be able to stand for 10 secs or so, during which you will oscillate sideways some before having to open your eyes or fall.
Degrading Vest is a bit trickier but still possible. Stand on both feet with eyes closed and spin around as fast as you can until you are dizzy, which results from the Vest system rapidly adjusting to the spin. When you stop, this causes a brief transient dizziness, during which if you immediately open your eyes and stand on one foot, you will be unable to balance until the dizziness subsides, usually after a few seconds. The effect is very brief but dramatic. Without Vis, balance is impaired, but without Vest, balance is completely impossible. People with severe damage to the Vest system are effectively immobilized. (It is possible to impair Vest less transiently, e.g. ingesting chemicals or physical head trauma, but I don’t recommend either. Both are surprisingly popular activities however, so it is likely you have witnessed or experienced this.)
SAT and DeSS: What is important about this experiment is that you can easily balance with both Vis and Vest, but not if either one is sufficiently degraded. This illustrates how layering involves SAT and DeSS. SAT is a speed-accuracy tradeoff (or law), and here Vest is fast (and inaccurate) while Vis is accurate (but slow). SAT is a crucial tradeoff throughout biology that we will discuss elsewhere in detail in several scenarios. For this task you are better off with Vest alone than Vis alone, and this is typical of layered systems. Lose a high application layer function like vision and you may have some other functions remaining but lose a lower layer function like VOR can be extremely debilitating. Loss of either in one leg balancing makes you quite fragile and quickly fall, whereas you easily stand with both intact.
Together, this is a layered Vis/Vest architecture in that both layers work in parallel to create a diversity-enabled sweet spot (DeSS) so that the resulting full control system is fast and accurate even though no individual parts are. We’ll explain SAT and DeSS in more detail, provide lots more examples, and explain how natural models of SAT in spiking neurons explains why such fragilities are unavoidable, but also normally not debilitating because of layering and DeSS. Layers, SAT, and DeSS are everywhere in biology, and are essential to control, learning, and even evolution. For now, note that the diversity in Vis and Vest enables a sweet spot where in combination they are fast and accurate when neither is both, but there is additionally a need for a layered architecture to produce the sweet spot, hence the “e” in DeSS.
A notable feature of this balancing task is the presence of oscillations before falling. In all 3 cases of standing on one leg with Vis, Vest, or Vis/Vest, most people’s body oscillates, largest in Vis only, more sustained in Vest only, but usually some even in Vis/Vest. Several key theorems (or laws) in control theory explain why this is unavoidable and ubiquitous in biology and technology, and this is an important aspect of our research.
Our next experiment simplifies balancing even further, focusing on just Vis and Vest, to allow more detailed exploration of laws, layers, and levels, in addition to SAT and DeSS. The simplest explores the vestibular-ocular reflex (VOR). This involves 2 steps. First, put your hand in front of your face so you can see the lines in the palm of your hand (or hold something with text). Then oscillate your hand in small sideways movements at increasing frequency until these lines start to blur (or you can’t read the text). This normally happens at around 2-3 Hz because the Vis system is again accurate but slow. Next hold your hand steady and shake your head “no” in small sideways motions until the palm lines again start to blur. For most people, the lines (and text) remain sharp (and readable) for much higher frequency motion, because Vest (via VOR) is fast (and the inaccuracy is less obvious than in the above balancing task).
VOR uses the same inner ear sensor as Vest and is the fastest reflex in the body, with eye muscles reacting to head motion in ~10ms. Vis actuates the same eye muscles as VOR but has delays at least 10x slower, which is a severe SAT. But a layered Vis/VOR has a DeSS that allows visual tracking of a moving object in the distance (Vis) despite running on rough terrain that causes rapid head motions (VOR). This would benefit in hunting large mobile prey animals, and humans have enhanced VOR systems over our nearest primate relatives. It also makes possible riding a bike down a twisting bumpy trail.
Our last experiment is balancing an extendible mechanical pointer upright on one finger (or hand), a human version of the classic inverted pendulum problem. This focuses on the Vis layer and the importance of the location of the Vis/Vest systems. The pointer needs to be long enough so that when fully extended it is easy to balance upright. (Unfortunately, many common visual disorders make this task impossible, so if you can’t balance at all, perhaps you can persuade a friend or family member to try the task so you can see the results. The most common problem is lack of stereopsis which causes a very predictable failure to balance. Closing one eye makes balance impossible for everyone.)
There are again 2 parts. First, shorten the pointer gradually and repeat the balancing task, always looking at the top tip of the pointer. As the pointer gets shorter, balancing becomes more difficult and ultimately impossible, for the simple reason that the instability becomes too fast for the slow Vis system to stabilize. With practice you can improve this somewhat, but short enough pointers are impossible for everyone. This combination of instability and delay is one of the most universal sources of fragilities in control systems and shortening the pointer (and thus increasing the instability) is one of the simplest illustrations. In contrast, varying the mass of a stick by orders of magnitude has little effect on balancing, though at extremes it can be hard to just hold, let alone balance.
For most people, their hand and the pointer will typically oscillate with larger magnitudes and frequencies as the pointer shortens, until the oscillations are so large that the pointer falls. This contrasts with an uncontrolled pointer that simply falls in one direction that depends on initial conditions. Note also that the oscillations occur roughly in the direction from the eyes to the pointer (so toward and away from you). Closing one eye makes balancing impossible. The same “laws” explain the oscillations here as well as in the above full balancing case, and a simple theorem that explains this is describe in detail in lots of papers and lectures available online.
For the 2nd part, extend the pointer as long as possible and keep the (physical) length fixed throughout the experiment, but now gradually move the focal point of vision (the measurement location your eye is looking at) down the pointer, occluding peripheral vision above this point using the other hand. Even modest lowering of the measurement point (to a level where the first part was still easy) makes balancing impossible, again with oscillations before falling. Thus a “bad” measurement location is even more severely limiting than the Vis delay and leads to unstable zeros in contrast to the increasingly unstable poles as the stick shortens. Unstable zeros cause even more fragility than delays, especially when combined with unstable poles, and thus will be important in our understanding of how to avoid fragilities. We’ll have reading, slides, and videos that go into this in much more detail.
Thus, in our original (one leg Vis/Vest) balance problem, it is essential that the Vis and Vest sensors be near the top of the body, and the head is a convenient location. Were either sensor to be located, say, near the midsection, balance would be impossible, and this would be true even with fast accurate sensors. Fortunately, the “fish parts” from which the Vis and Vest systems evolved were already in the head. There are other examples, such as the recurrent laryngeal nerve (RLN), where the wiring in fish was less fortunate for us, leading to obviously suboptimal circuitry.
It is easy to model and analyze this pointer balancing problem, as it is essentially the cart and pendulum control problem that is used as a standard educational demo plus some limits due to human sensorimotor control. There are two equilibria, down and up. (To get the down case, lightly hold one end of pointer between your fingers and let it dangle below your hand.) The down case is the usual pendulum which oscillates at a frequency that depends on the length and is robust enough that it was used in early clocks to tell time. This depends on standard mechanics and gravity, and speed of light is fast enough that its delay from pointer to eye is negligible. These are all familiar “laws” in physics.
The up case in contrast is unstable and fragile and requires active control, and many people cannot learn to balance the pointer under any conditions. As described above it can be easily made impossible, even for experts, by adjusting the length or the sensing. There are less familiar but equally constraining “laws” of control that explain this and have been the focus of much of our research.
To explain the oscillations and their directions, and the failures at low lengths and measurement locations, as well as those in the one leg balancing, requires an additional law from control theory that was not previously applied to sensorimotor control. This law also explains other cryptic oscillations in biology (e.g. glycolytic). This highlights that there can be “bad plants” where control is necessarily arbitrarily fragile, and in general that robustness has strict conservation laws that are essential to understanding the examples we are considering here, as well as those throughout the course. In this case, these laws also limit what can be learned, but we will be looking for other “laws” of learning. A central question is whether there are additional limits to learning that are intrinsic and not just an accident of the data taken.
Returning to the Vis/Vest layered control for one leg balancing, we can see how the Vis/VOR hand/head motion stabilization control highlights the SAT and DeSS in the Vis and Vest layers. Then the pointer balancing problem and the right laws from control theory show how oscillations and falling depend on the instability of balancing plus the delays in Vis. The one leg balance problem combines the Vis/Vest layering and the balancing, and thus has new laws, SAT, and DeSS that explain all the patterns we see, including oscillations and failures when either Vis or Vest are degraded. Note that pointer balancing is impossible at any length with just one eye, whereas one leg plus one eye balancing is hard but possible. See if you can guess why, and we’ll discuss this later, as well as a trick that allows stick balancing with one eye.
What these experiments collectively illustrate are certain “universals” of complex control systems, including layering for DeSS to overcome hardware SATs, and new laws from control theory to explain oscillations and crashes and their dependencies on problem conditions. More subtle and significant is how these tools combine to create a platform for a more rigorous systems biology and neuroscience, and hopefully a theoretical foundation for robust learning. Lectures online use these simple experiments as a starting point for explaining this platform, and also illustrate it in other applications in biology, medicine, and technology.
Levels are also important: These extreme diversities in function, behavior, and performance across layers such as Vis/Vest/Prop, are matched by equally extreme diversity in the lower level nerves that implement them. While less essential to this course than the laws/layers experiments above, the orthogonal notion of levels is ultimately as essential to understanding biking, balance, sensorimotor control, and learning in general. The Vis/Vest layers are all at the system level and are implemented in a lower nerve level. So, in addition to Vis/Vest layering, there is System/Nerve levels for both Vis and Vest systems, and this is essential to understanding the origins of the extreme SAT we see in Vis versus Vest.
There are many orders of magnitude diversity in nerve size (thus cost to build and maintain) and composition (e.g. axon size and numbers, and thus latency and quantization), as well muscle composition (slow to fast twitch), and in other brain components engaged in all the above experiments. In sensorimotor control more broadly, the research challenge is to make sense of the apparently bewildering complexity of both sensorimotor behavior and its neural substrate. One large and central line of existing research is called Speed Accuracy Tradeoffs (SAT).
Fitts’ Law is the canonical example of SAT and is the single most studied topic from basic neuroscience to human factors engineering. In most motor tasks (e.g. reaching, throwing, riding a mountain bike down a twisting rocky trail, etc) there are a variety of tradeoffs between the speed and accuracy of movement, and can include distance traveled and time allowed, as well as preparation time, advanced warning, unmeasured disturbances, delays, instabilities, etc.
Basic Fitts involves reaching for a target of width W over a distance D while trying to minimize the time T, and surprisingly, T scales with the log(D/W). Other analogous logarithmic scaling laws pervade biology and neuroscience (with names like Weber-Fechner, Bohr, Ricco, …). Most simply Fitts means that the time to move a distance D (with a fixed error) scales not linearly with D (which would be true if speed were uniform) but much more favorably with log(D/W). We showed that one absolutely essential element to get the favorable log scaling is a “diversity-enabled sweet spot” (DeSS) in the muscles controlling movement [Nakahira et al]. Muscles themselves have a SAT ranging from “slow twitch” which are accurate to stronger “fast twitch” which are not. Any uniform muscle can only produce a linear Fitts law, and exactly the kind of accurate versus fast mix of muscle types seen in real physiology is necessary and sufficient to get the much more favorable log scaling seen in Fitts experiments.
Both Fitts law and muscle diversity are extremely well studied (particularly in human factors and sports medicine research) and understood but the connection had not been explored, let alone rigorously established. While both the experimental and theory literature is vast, there has been until recently no satisfactory theoretical explanation for Fitts or other such log laws (though obviously the theorists involved would vigorously dispute this, one source of the bimodal response to this work). A somewhat remote exception is Noah Olsman, who working with Lea Goentoro has a PNAS paper on log laws at the protein level.
We used very standard, and thus hopefully noncontroversial, nerve and muscle component models throughout and derived Fitts law using basic extensions to control theory. But Fitts is in retrospect an almost trivial example of log scalings and DeSS, and we have greatly extended SAT and DeSS throughout the nervous system and its behaviors, and we are just beginning to explore this vast new frontier. While we are not experimentalists, this subject has such huge gaping holes that it is easy to use cheap video gaming platforms to create novel experiments with greatly expanded versions of Fitts laws with rigorous theory.
DeSSes are everywhere in both our biology and our technologies but are generally hidden (e.g. virtual memory hierarchies in computers, CPU/GPU/FPGA/ASIC hardware, etc.). An obvious one not hidden is human transportation, where even a trivial mix of walking, driving, and flying greatly extends Fitts-like DeSS for reaching. Flying (F) is fast but is (mostly) limited to airports so by itself is inaccurate, walking (W) is much more accurate and slower, and driving (D) is in between. Individually the SATs are severe and limiting but combining them in the right order (e.g WDWFWDW) gives us global mobility at nearly the speed of jets and the accuracy of walking. Combining them badly can be slow and inaccurate and even catastrophic. We perform this combination to create a DeSS consciously and manually, with some tech assistance in planning and timing routes, but in the nervous system it is largely automatic and unconscious, and the wild diversity in the nervous system implementation (e.g. nerve size and composition) is hidden and less emphasized in neuroscience research.
An important recent innovation was in matching our new theory with realistic models of spiking neurons (which motivated it), and then mapping the SAT at the nerve level (e.g. in terms of axon diameters and numbers, and nerve lengths) to the SAT at the behavior or functional level (e.g. riding a bike down a bumpy twisting trail without crashing, balancing on one leg, etc), and then making video games and other demos that noninvasively demonstrate the theory in all its richness. Our new control theory results that add layers/levels to the known laws have appeared in control conferences and been vetted by experts and are significant advances in doing robust control over communications and computing that is layered, quantized, and delayed.
But we are just now doing (hopefully) final revisions on papers for science journals, and I’ll post the latest. Surprisingly, there was previously essentially no research quantitatively connecting SATs at the spiking neuron level with SATs in system behavior, not just in Fitts related task but anywhere. Based on our presentations and posters at neuroscience meetings so far, and reviews of the papers, this work is a major shock and gets extremely bimodal reactions from neuroscientists. (A famous neuroscientist complained that the whole idea that the vestibular system is “fast but inaccurate” compared to vision is just total nonsense and the emphasis on diversity seems political.) Fortunately, Terry Sejnowski is a major mainstream neuroscientist and is our main collaborator on this work, and the positive reviews appear to be favorably inclining editors to at least consider this.
As a final thought experiment note that in all the tasks above there is sensing (e.g. vision, vestibular, proprioception) that results in some action due to actuators, in these cases muscles perhaps working via steering wheels and brakes. In between is complex decision and control implemented in communication and computing networks. In biology and technology there are limits on control that can arise in any of these components as well as their interaction. In biking and balancing we emphasized the SAT in sensing, particularly in Vis and Vest, and in Fitts we emphasized the SAT in muscles. We largely avoided communications and computing SATs by lumping them into the sensing and actuation, but in general these must also be treated explicitly, and we’ll need tools to do that. What is fairly universal and important, but not emphasized here, is the asymmetries between SAT and other tradeoffs in sensing vs. actuation and computing vs. communications. Computing and memory are usually easier, cheaper, and less constrained than communications, and sensing is much easier and cheaper than actuation. Also, accuracy is typically cheaper than speed in all components.
In the walk/drive/fly travel example, sensing, computing, and communications are cheap and efficient and essentially all the limitations are due to actuation. While biking uses many muscles, in downhill biking these work via just two main actuators, steering and braking, with body adjustment adding some additional actuation. In contrast, vision senses the trail in comparatively very high dimensions. The “look down” pointer balancing severely and somewhat artificially constrains vision and is easily mitigated by allowing more natural use of vision. The delay involved in the short pointer problem however comes mostly from vision, and there is no simple way to change this. As a thought experiment, notice that in most of your daily activity the dimensionality of what is sensed is vastly larger than what can be directly actuated. Vision can remotely sense well beyond what can be touched. Even more extreme are some of the games that ML excels at compared to humans (e.g. chess and go) in that sensing of the game state is perfect, there are no constraints on communication other than those that arise within computing systems, and the main constraint is on the allowed moves. So, these games are limited primarily by actuation and increasingly less so by computing, and not at all by sensing. Perfect state measurement is also a simplifying assumption made often in control theory, and one we will also use frequently, but it will be important to understand the limitations on this simplification.
What about cognitive tasks that interfere with balancing? There is a literature on cognitive motor dual task interference that includes studies showing that cognitive tasks can interfere with balance, and this is aggravated by aging. This is in addition to the even larger literature on interference between cognitive tasks. But I don’t know either well and connecting with this research could be interesting. One of our collaborators (who happens to be in high school) is adding cognitive tasks to the biking game to see what does and doesn’t interfere, and I’m sure she’d be eager to have more people to talk to about this if anyone is interested.
From John Doyle: reviewing layers and levels, trails and bumps, brains and nerves.
We’ve focused on the mountain biking example because it is familiar to everyone, extends traditional Fitts tasks simply but significantly, and can be simulated safely and cheaply using standard video gaming platforms. We’ll now review the main ideas this motivates and go a bit further in considering additional layers including the rider and bike that aren’t in the game version. In real and simulated biking, vision is used to see the trail ahead, and this advanced warning is used to plan a route that tracks the trail. While this is all an enormously complicated process, we’ll initially think of it as a single integrated function involving vision, planning, and tracking. This can be thought of as a “trail app” or task that suitably trained and equipped humans can learn and implement, and we’ll call the nervous system components that perform the task the “trail layer,” recognizing that much of this layer is only partially understood. Overall, this layer is relatively slow but accurate, as even large turns in the trail can be tracked with small error provided there is enough advanced warning relative to speed, and the bumps are adequately handled.
The bumps in the trail (also easily simulated using motor torques in a gaming steering wheel) and the stabilization of the unstable bike/body dynamics are handled by a separate reflex layer that is unconscious and automatic and has necessarily delayed reactions to unseen bumps, in which even small disturbances can easily result in catastrophically large errors and crashes. These reflexes in the “bumps layer” are relatively inaccurate but fast and must deal with the dynamics of the bumps, the bike, and the rider’s body and are in addition to the VOR (vestibular ocular reflex) which is stabilizing vision despite head motion. This layered architecture allows trained riders to simultaneously multiplex the trail and bump tasks, because they are implemented in separate but cooperating trail and bump layers. (There are other layers that multiplex myriad tasks like maintaining internal homeostasis despite physiological demands.)
Layering is much more than mere parallelism and means the slow accurate trail layer can use highly virtualized and stable, predictable models because the fast, inaccurate bumps layer automatically controls the wildly unstable and unpredictable lower layer including body and bike. And good plans and tracking in the trail layer make reflexive control in the bumps layer easier and vice versa. This combination of layers is an example of a diversity-enabled sweet spot (DeSS) in SATs across functional layers and is complementary to the simpler SAT DeSS in Fitts within the muscle component.
With enough advanced warning and resources and functioning reflexes, we can be almost perfectly robust, and at the opposite extreme, almost infinitely fragile if some layer is compromised or the mix of speed, turns, and bumps exceeds the rider’s capability. Good athletes also multiplex these tasks extremely well with modest training, as it exploits existing architectural features humans have evolved in becoming top predators. In contrast, adding a task like texting would be catastrophic as we are not evolved to multiplex this with trails.
These extreme diversities in SAT for function, behavior, and performance are appropriately matched by equally extreme diversity in the high level brain systems (e.g. cortex vs. spinal cord) that would be engaged in such a task. What is needed is a theory that mechanistically explains how multiple diverse layers (e.g. trails and bumps) within this high level are effectively multiplexed for complex tasks, and further how this functional level is implemented in the equally diverse spiking neuron level. Both control theory and neuroscience need an upgrade to achieve this, which we now have.
Both trail and bumps layers are within the functional level made up of brain and nervous system components, to distinguish it from the nerve (and muscle) level that implement both layers in spiking neurons. The Fitts reaching task has only a trail-like layer with no bumps. Thus, our DeSS theory for Fitts has levels but no layers. It involves just one functional reaching layer and level, implemented in a lower muscle level, and the diversity in muscles explains the DeSS in reaching. In contrast, the trails and bumps are separate but coordinated layers, and both are in the same functional level, which is in turn implemented in the lower level nerves and muscles. Conventional control theory poorly addresses both the layers and levels in such problems, as the different layers involves distributed control, and the lower nerve level implementation has severe SAT due to quantization and delay in spiking neurons.
While we are primarily theorists, and emphatically not experimentalists, we have worked with a variety of collaborators to build a simple video game with a twisting virtual trail and “bumps” generated by motor torques in the steering wheel used to follow the trail. We have received enormous help in developing the game from other grad students, some undergraduates, and even high school students. This game mimics the plan+reflex challenge of the real thing remarkably well without the dangers (that would preclude IRB approval). Our new theory matches preliminary data extremely well and we will continue to develop both the game (adding additional tasks) to further explore the theory. As far as we know, there currently isn’t anything remotely comparable to this theory or its applications being done anywhere else in neuroscience that directly addresses the layered architecture, the speed/cost/accuracy tradeoffs in the performance versus the hardware, or the combination of SLSDNQD sensing, communications, computing, and actuation. In fact, the little that does exist explores at most pairwise tradeoffs in these elements.
That the optimal nerves for implementing trail and reflex layers have extreme DeSS both between and within nerves, is one of the striking predictions of the theory that matches both data and physiology. Oversimplifying somewhat, the key tradeoff is between high bandwidth (lots of small axons) or low latency (a few large axons), and the trail and bumps layers are dominated by the former and latter, respectively, both in theory and reality. The theory also predicts that an optimal architecture and components would allow trained riders to handle trails and bumps together with an error that is roughly equal in size to the sum of the errors when the tasks are performed separately, and most subjects quickly learn to achieve this in practice though the rate of learning and final error levels vary significantly between subjects, for reasons we hope to explore.
Thus, the theory captures both the essentials of layering (effective multiplexing of trails and bumps) and levels (extreme DeSS in neural implementations), in a way that had not been done before. The long-term goal of our research is to create a rigorous theoretical systems neuroscience that would be a foundation for studying sensorimotor control, but also hopefully for cognition and decision-making as well as homeostatic regulation. The proximal goal has been to more deeply understand layered plan+reflex control in general as an entire system and particularly the patterns of massive feedbacks both within visual pathways and between visual areas and motor control of eye muscles and attention. Now we want to also focus on the interplay of learning and layering.
These issues extend down to the cellular level in biology and outward to our most advanced network technologies, and our research plus the much larger effort on System Level Synthesis (SLS) finally address these challenges in a coherent way.
Laws, layers, levels in active/passive/lossless
In the biking example, the Vis/Vest layers are all active control, and our models of spiking neural networks treat them as essentially digital packet switched networks (though the results don’t depend crucially on this, just that the theory allows for it). But there are digital/active/passive/lossless layers in sensorimotor control, with passive damping mechanism in the bike and rider, and nearly lossless rolling on wheels. An important and universal point is that the more lossless we make the lowest layer the harder control (and learning) becomes at the higher layers. Human’s upright running is much more efficient than chimps at long distances, but is more fragile and needs more complex control, particularly in the Vest system. Just adding a bike alone is more efficient than running but is even more fragile, requires additional learning, and makes crashes much more severe. Further streamlining, fairing, and refining the bike for greater efficiency will make it faster on ideal surfaces but impossible to ride on twisting bumpy trails. This tradeoff between low layer efficiency and higher layer robust control and learning is truly universal and needs more systematic attention in a theory of sensorimotor control as well as sustainable CPS (cyber-physical systems) infrastructure. We have used robust efficiency tradeoffs to explain previously cryptic glycolytic oscillations and patterns of heart rate variability, but “full stack” design addressing such robustness efficiency tradeoffs in general is an important research direction.
The physics of levels
There is a completely different issue of active/passive/lossless (A/P/L) levels, which we have not discussed and is a major source of confusion, partly because science has so far only studied levels (not layers), and in our thinking, inadequately, whereas engineering studies both but rarely clarifies the distinction. Levels will not be a major focus here, compared with layers, but we’ll review the distinction to clarify what can be a source of confusion.
The A/P/L layers are all layers of macroscopic systems, of roughly comparable size. As levels, active and passive are simply different ways of viewing the same macro components, but physics tells us that their micro descriptions are lossless (and quantum, though we’ll defer that issue for now). Thus, a central mystery is how our deterministic digital systems level can be built from a stochastic analog circuit level, which in turn must have a passive implementation level, all of which is microscopically lossless and deterministic (classically).
The digital to active to passive to lossless (all macro) levels are the subject of engineering, and are reasonably well understood, if less coherently than is needed for a more complete theory. The passive macro to lossless micro levels is the subject of classical statistical mechanics, but there is no unified theory, and what pieces do exist are inadequate. This all seems fixable and we have made some initial progress. We have developed an alternative control-theoretic approach to classical stat mech that we believe is both more rigorous and relevant to engineering and biology, and already connects to macro active levels.
1. Sandberg , Delvenne, Doyle (2011), On Lossless Approximations, the Fluctuation-Dissipation Theorem, and Limitations of Measurements, IEEE Trans Auto Control, Feb 2011
2. Huo, Doyle (2019), Measurement back action and a classical uncertainty principle: Heisenberg meets Kalman, American Control Conference, 2019.
I mention this levels topic primarily to distinguish it from the layer topics emphasized above. Note also that our sensorimotor levels story from brain/spine level to nerve level is all within the digital or active levels so stat mech issues don’t arise. What is perhaps relevant here is how the laws of Turing, Bode, and Shannon can be unified and also appropriately generalized across the digital/active/passive/lossless layering and macro levels, particularly to included learning, and deferring for now the macro passive to micro lossless issues addressed in [1,2]
While fragments of this challenge can be explained with existing control theory, there currently isn’t anything remotely comparable to SLS (System Level Synthesis) or its applications, that directly addresses the layered architecture, the speed/cost/accuracy tradeoffs in the performance versus the hardware, or the combination of SLSDNQD (sparse, local, saturating, delayed, noisy, quantized, distributed). It also looks ideally suited to combine learning with control, so this will be a focus here.
Finally, all these issues extend down to the cellular level in biology and outward to our most advanced network technologies. For the first time, we have a coherent theoretical framework that addresses all aspects of this problem:
3. J. Anderson, J. C. Doyle, S. H. Low, and N. Matni, System Level Synthesis, Annual Reviews in Control, 2019.
4. Y.-S. Wang, N. Matni, and J. C. Doyle, Separable and Localized System Level Synthesis for Large-Scale Systems , IEEE Transactions on Automatic Control, 2018.
5. Y.-S. Wang, N. Matni, and J. C. Doyle, A System Level Approach to Controller Synthesis , IEEE Transactions on Automatic Control, 2019
and have worked with neuroscience specialists and experimentalists to prove the theory works in practice.
Central to our theory is that layered architectures enable not only diversity, but diversity enabled sweet spots (DeSS) that give systems with properties (e.g. both speed and accuracy) that no component alone has. The latest versions of these papers will be available.
6. Y Nakahira, Q Liu, N Bernat, T Sejnowski, J Doyle, Theoretical foundations for layered architectures and speed-accuracy tradeoffs in sensorimotor control, 2019 American Control Conference (ACC), 809-814 (see also arXiv:1909.08601)
7. Q Liu, Y Nakahira, A Mohideen, A Dai, S Choi, A Pan, DM Ho, JC Doyle, Experimental and educational platforms for studying architecture and tradeoffs in human sensorimotor control, 2019 American Control Conference (ACC), 483-488 (see also arXiv:1811.00738)
8. Y Nakahira, Q Liu, T Sejnowski, J Doyle, Fitts' Law for speed-accuracy trade-off describes a diversity-enabled sweet spot in sensorimotor control, arXiv:1906.00905
9. N Bernat, J Chen, N Matni, J Doyle, The driver and the engineer: Reinforcement learning and robust control, 2020 American Control Conference.
We can use the biking example to illustrate further aspects of our “full stack” layered architecture approach as well as the role of model-based methods, all enabled by SLS. Mountain biking illustrates the importance of passive and lossless actuation below the (active) reflex layer, a design challenge poorly addressed by previous methods. Bike wheels greatly reduce frictional drag (versus unwheeled locomotion) and allow for much greater speeds with much less effort, so are nearly lossless energetically compared with the rest of the system. The ride is further greatly improved with the addition of passive damping systems, present in advanced mountain bikes (and in the legs of the rider).
The proper layering of passive and lossless bike mechanisms together with learned active sensorimotor plan/track/reflex layers can greatly improve the performance of the bike plus rider, and we are currently expanding the SLS theory to allow for explicit and optimal designs of these “lower layers” of lossless\passive in addition to the existing theory for the trails and bumps layers. This is immediately relevant to legged motion where humans and animals outperform robots, in part because biology has more sophisticated layered controllers of the type this theory has. It is also relevant to more general physical process design, which always has efficiency as a primary and often poorly achieved objective. Note that traditionally, control has poorly addressed this full stack design problem, as constraints on controllers such as passive and lossless were intractable. This is remarkably parallel to the SLS story for SLSDNQD constraints which were also deemed intractable. While much remains to be done, it appears likely that SLS and new variants may provide a unified theory of both active/passive/lossless layering and SLSDNQD constraints.
Here we’ll focus primarily on the digital layers, the role of security and immune systems, and adding learning to control, but a major theme is that there are hard limits or laws constraining what is possible that depend on the lower layers and can be aggravated by improvements in these layers. Indeed, when considering the full stack design problem, the most fundamental tradeoff is between robustness and efficiency, and SAT is a refinement of the robustness dimension.
From John Doyle: the layered architecture of fragility and (un)sustainability
Acute vs chronic, and accident vs architecture
Some distinctions that seem important but are poorly formalized are acute vs chronic, and accident vs systemic. Consider the “forensic” accident analysis after an auto or plane crash. A single, isolated crash or even a large error might be determined to have been pure accident, “bad luck,” an “act of god,” etc. and this will prompt neither vehicle redesign nor driver/pilot retraining. If similar crashes are recurring, then this chronic condition will suggest these are not mere accidents but have a systemic aspect that needs changes to vehicle or pilot. We are going to focus on fragilities in complex networks that are chronic and the most systemic but recognize that classifying errors or crashes along these axes is itself a challenge.
Twin chronic and systemic fragilities in complex networks are hidden consequences (bowtie) and infectious hijacking (hourglass). These will not be the only or even primary kinds of fragilities that will be our ultimate focus but understanding how our CPS infrastructure creates a range of fragilities is essential to making them more sustainable.
Learning has the potential to greatly aggravate these fragilities but ideally mitigate them, so we need to understand their basis and origin. This “rant” on sustainability in complex networks aims to raise new questions about tradeoffs, diversity, constraints, and fragility, but in familiar contexts. The corresponding new (and still incomplete) answers require lots of pictures, diagrams, and math, expanded on elsewhere. This text is aimed to be simple and accessible and motivate the theory to come.
Protocols and their role in layered architectures have arguably been the primary enablers in the proliferation of our most unsustainable and dangerous technologies, and their hijacking enables the pathogens that threaten medical pandemics as well as the malware in cyberattacks. Improved protocols in technology and medicine are also our only hope for health and sustainability. An intrinsic feature of protocols in technology and biology is that their details are hidden and cryptic in normal operation, and thus their role in layered architectures are both critical and poorly understood. This is true from molecular protocols in biology to the protocols governing our global (and unsustainable) economy and everything in between.
As a concrete example, you are reading this via layered networking protocols (e.g. TCP, IP, HTTP, SMTP, DNS, DHCP, VPN, …) using a layered English grammar architecture (phonemes\words\syntax\semantics) running on an even more complex layered nervous system, all of which is largely hidden (unconscious, automatic, implicit, etc.) and must necessarily be so to be functional. (We’ll use the shorthand hi/low and low\hi for layers interchangeably.) We act (flip switches, drive, fly, eat, drink, shower, flush, heat, cool, etc) in ways that seem effective to the user but are profoundly unsustainable and the sources and destinations of everything remains largely hidden, and temporarily ignorable. Even experts individually typically know only tiny slices through how any of this works in any detail.
Protocol-based layered architectures, from the most ancient microbes, to the earliest agriculture and cities, to the most modern cybertechnologies, by evolution and design hide their ecological and resource consequences. Our only hope seems to be to leverage their extraordinary evolvability not primarily to give consumers ever greater choices but to make all choices automatically sustainable in ways that will remain largely hidden. Tweaking components of these systems is still important but is likely to have modest impact without a more comprehensive architectural upgrade. For example, extensively deploying renewables in the current “dumb” power grid will create nonstop blackouts before they provide much greater sustainability. Slapping learning on top might help nominal behavior under ideal circumstances, but risks making cascading failures worse.
We have a coherent research and deployment path to a sustainable future energy “smartgrid” but much less for other systems (e.g. transportation, food, medicine, housing). There are consistent architectural features necessary to make them sustainable, and consistent homeostatic regulation strategies to avoid outages, but much less of an R&D plan to make this happen beyond the electric grid.
What we urgently need to create a more sustainable and livable world is not just better parts (from molecules to batteries to turbines) but better protocols and their architectures. Technology must become more like biology, not in parts but in architecture. What does that mean in practice and in theory? We need a theory of complex network architecture that treats control, learning, evolution, and evolvability in a coherent manner, and can inform practical design.
Layered architectures in evolution, learning, and control
Fortunately, some features of layered architectures are very familiar, particularly universal "bowties and hourglasses" that appear in complex highly evolved systems at every scale and context. In both bowties and hourglasses two outer deconstrained layers, with very diverse components that are evolvable and even swappable, are linked in the middle via a narrow, highly constrained knot/waist with little diversity or evolvability. We call this “constraints that deconstrain” (as in Gerhart and Kirschner). The terminology of bowties and hourglasses is not standard and can be confusing, but the distinction is useful and important. This will be qualitative discussion of motivating examples with technical details elsewhere.
Diversity is the aspect of layered architectures that is most familiar. Diverse genes and proteins are linked via highly conserved (and minimally diverse) transcription-translation protocols. In metabolism, diverse carbon sources and biological molecules are linked via a thin “knot” of a few metabolic carriers and precursors. Diverse electric power sources and user appliances are linked in a bowtie via standard knot protocols (e.g. 110v 60Hz) in power grids. These examples all involve the flow of materials and energy and with respect to diversity have a bowtie shape, with diverse sources and products at the edges and highly conserved and less diverse “knots” in the middle.
An hourglass is used to describe the shape of layered communication and computing systems required to control bowties. Diverse app software runs on diverse hardware in an hourglass linked via less diverse “waist” operating systems (OS) in computers and their networks. Humans have diverse skills and memes and diverse tools, linked in an hourglass by shared languages and a poorly understood brain OS. Humans have a layered language of phonemes\words\syntax\semantics with few phonemes, much more diverse words, and extremely diverse meanings, but less diverse syntax. Genes, apps, memes, words, technologies, and tools are highly modular and swappable, massively accelerating evolvability beyond what’s possible with only the slow accumulation of small innovations. We’ll discuss a few of these in a bit more detail.
In the metabolism bowtie, extremely diverse sources (e.g. of carbon) are linked by via a few universal metabolic carriers (ATP, NADH,…) and precursors (pyruvate, G6P, F6P, PEP,…) to extremely diverse building blocks (amino acids, nucleic acids, lipids, …) and products (enzymes, ribosomes, chromosomes, membranes, etc) . While there are also diverse enzymes that catalyze these reactions, the core protocols consisting of the precursor/carrier “knot” of this bowtie and the wiring diagram that interconnects them are universal across all of biology. Biology is also massively autocatalytic, with enormous feedbacks of energy and materials, far more than our technologies, and this is an essential element of sustainability. Autocatalysis is essential for efficiency (i.e. approximately lossless) but also makes homeostatic regulation much harder in a way we can make precise, and this will be a challenge in sustainable technologies.
Similarly, diverse and evolvable genes control a universal, conserved “knot” of core transcription and translation protocols to produce diverse proteins, again with massive autocatalysis. Both metabolites and genes are promiscuously exchanged in the bacterial biosphere, a feature that together with the protocol universality are exploited by phage and other parasites. This hijacking can be unsustainable and can lead to the death of the host or the collapse of ecosystems, even when this is not optimal for the parasite. More favorably, less well understood “diplomatic protocols” between organisms facilitate the thriving of diverse ecosystems.
Every aspect of this story has parallels in technology, but the hijacking and unsustainability now threaten the global ecosystem. For simplicity and concreteness, consider modern energy technologies and particularly the electric grid. Diversely located and fueled generation is transmitted and distributed by a universal set of protocols, with a simple universal 110V 60Hz (in the US) plug interface that then supplies an even more diverse set of downstream user technologies, including both manufacturers and consumers (which form yet another supply chain bowtie). The universal energy “knot” has enabled explosive evolution and deployment of diverse supply and demand technologies, but also hides from users the ultimate effects of those technologies on resource depletion and waste production. The use of fossil fuels in energy and transportation is profoundly unsustainable in ways I assume is unnecessary to belabor for this audience. We flip a switch and get instant power with literally no sign whatsoever of the upstream and downstream consequences even when these are increasingly catastrophic.
Analogous bowties, protocols, and the resulting hidden unsustainability are present in all forms of transportation and the production, consumption, and waste associated with food, water, consumer goods, military equipment and operations, and all modern economic activity. Again, the very nature of the layered bowtie architecture means that essentially all the negative unsustainability consequences are hidden in normal operation. We flip, drive, eat, drink, shower, flush, heat, cool, etc and the sources and destinations of everything remains largely hidden, and ignorable, even apparently deniable. This is not an accidental feature of layered architectures but their essence and the main enabler of both their success and unavoidable unsustainability. We use “virtualization” as the label for this aspect of layered architectures, to distinguish our use of the architectures from its consequences in the “real” world.
Simply removing all layered bowtie technological architectures would reset us to at least stone age, as cities and their associated slave and animal-based agriculture were the first architectures to employ layering in an essential way on a large scale. Our only choice is to exploit layered architectures potential for extreme efficiency and robustness by adding layered “hourglass” control architectures for homeostatic regulation of our “bowtie” produce/consume/waste systems for energy, transport, manufacturing, food, etc. Our cells and bodies already use layered control architectures to maintain homeostasis, and the average human produces 100 watts at 25% efficiency, thus needs 400 watts of energy from food on average (need to check this!!!!). In contrast, the US consumes 4 trillion watts (4 terawatts) of just electric energy on average, which is more than 10K watts per person. (Need to check all of this. BTW, food waste in all forms dwarfs actual food consumption in every dimension, hard to quantify?)
Fortunately, there is abundant renewable wind and solar power to easily supply all our energy needs, and we now largely understand the technological challenges to the deployment of a completely renewable energy system. The principle challenge is not cheaply meeting the average energy production requirements even in the tens of terawatts, but homeostatic regulation of the unavoidably uncertain and fluctuating supply that renewables provide. Fortunately, we now largely understand how to do this using layered control architectures analogous to what the body uses for allostasis and homeostasis, but its practical deployment will be challenging (though perhaps less than creating the political will to do anything at all). Like the body, this future “smartgrid” will be not only renewable but also quite naturally “reusable.” That is, the supply, transmission, and distribution components for electricity naturally age gracefully, unlike our current tech to contain and transport other consumables. The only plausible sustainable future is a completely renewable and reusable smartgrid, plus a shift of all our other architectures to be similarly renewable and reusable, much of it via electrification. Both biology and the future smartgrid provide templates for how this could be done.
Our most canonical layered “hourglass” control architecture is the Internet’s, and it will evolve to provide some of the communication and control elements of our future “smartgrid.” (We use “hourglass” to describe the layering of control, simply as a convention to distinguish it from bowties that describe the flow of materials, energy, or information.) Put simply and concretely, a bewilderingly diverse “apps” layer sits atop a thin “waist” universal TCP/IP layer (P is for protocol), which in turn sits on a nearly as bewilderingly diverse hardware layer. (This grossly oversimplifies the modern Internet but captures the essence we need.) TCP/IP is analogous to the operating system (OS) on a PC or smartphone, and it manages and “virtualizes” the hardware resources. Thus, both apps and hardware need only talk to the OS, and rarely directly with each other. This facilitates evolution, including promiscuous swapping analogous to horizontal gene transfer (HGT) in bacteria, with a similar fragility to hijacking by pathogens, in this case called “malware” instead of software. It also means that almost no one need know the details of the architecture in its entirety and can usually focus on one part within one layer.
Layered architectures also create the opportunities for winner-take-all deployment of either a new OS (Microsoft in the 80s) or new “waists” atop the “OS” with familiar examples such as search (Google), consumer (Amazon), social (Facebook), and even more integrated functionality (WeChat). The eukaryote cell architecture created a new waist on top of the basic bacterial cell, as did metazoan development, nervous systems, and societies (all of which have bowties as well). Languages have layered grammars with semantics, syntax, and words/phonemes playing the roles of apps, OS, and hardware, respectively, which similarly facilitate evolution, swapping, and hijacking. Our biggest human problem now is that our unsustainable technologies are aggravated by our belief in “memes” that are viral, false, and dangerous (including that our technologies are not unsustainable).
What we must do
Basically, everything must become like the best of biology and the Internet and follow our plans for a future smartgrid. Electrify everything obviously but also make the other technologies more autocatalytic, more renewable and reusable, and in terms we will explore below, more lossless. This will require profound changes in the architectures of transportation, food, water, waste, and all our supply chains. Unfortunately, the virtualization that is an essential feature of layered architectures delays and diffuses their deleterious impacts until it is too late to avoid catastrophe. And we currently have minimal allo- and homeo- static regulation of any of our myriad bowties and making them more sustainable will make this far more challenging. The technical understanding of these issues is limited to a handful of experts (though Caltech is relatively well positioned in this subject despite its small size).
So even experts who recognize our current unsustainability have no coherent research plan to understand what is needed, let alone a development path to fix it, with perhaps the exception of smartgrid experts. This too is a feature of layering and virtualization, it hides the details from everyone, include most experts, most of whom are necessarily focused on technology within one layer (and often within one level). And experts who do understand all the details of one architecture (e.g. smartgrid or internet or cells or brains ) may have novel insights into others (brains or cells or internet or smartgrid) but can find the details of the “apps” and “OS” and “hardware” involved to be bewilderingly complex. And we don’t even know exactly what the “OS” is in, say, the brain or healthy societies (for which there are no large-scale human examples). Worse, science is currently dominated by some viral “memes” around complexity and networks that explicitly deny that architecture is relevant, and policy is dominated by equally bad “memes” denying that sustainability is a problem. (Sorry, that is sounding like a rant, even if it is true.)
Some good news
The good news is that in the last few years we finally have many of elements of a coherent theory of layered architectures that goes well beyond [2] and have begun applying it successfully to canonical case studies such as smartgrid, sensorimotor control in mammals, micriobiomes, cancer and wound healing, and animal societies, and in earlier forms to cell biology and medical physiology. However, all of these were relatively simple initial cases, and all need much more development. It has not been applied systematically to agriculture, global supply chains, water, waste, human societies and organizations, markets and economics, etc. but these are all examples of natural but challenging next steps.
Laws, layers, levels, SATs, and DeSS in biology, computing, memories, and other technologies
Likely the most familiar layered architecture for our readers is apps/OS/HW hourglass in our phones and computers, and their extensions to networks (as described above), so this is a convenient example to expand on. Within the HW layer, the memory hierarchy can also be thought of as layers with register\cache\RAM\disk\tape having an extreme diversity in speed, size, and cost. (We’ll use the shorthand hi/low and low\hi interchangeably.) Memory also illustrates “levels” with each layer being implemented in lower level components, such as circuits and transistors. Of course, the apps and OS layers have sublayers and levels, and so on. Layered architectures are everywhere in technology and biology, but virtualization means that details are often hidden from nonexperts. We will adopt an admittedly greatly oversimplified view to highlight broad similarities and differences and encourage experts to add additional details.
Note that in this usage layers and levels are essentially orthogonal and complementary concepts but there is no standard terminology, so we are suggesting one. The notion of “levels” is standard in physics (classical/quantum) and biology (organisms/cells/molecules), but “layers” is emphatically not, and is taken from its usage in networking and engineering.
An ideal memory would be fast, large, and cheap, but no such individual component exists. This tradeoff can be loosely thought of as a law, but one that depends on available technology in addition to more fundamental laws of physics. Here we use “law” and tradeoff broadly (and somewhat loosely) to describe constraints, including legal or regulatory, on what components are available. In engineering, our favorite “laws” are due to, e.g. Bode, Shannon, and Turing, and in science to Newton, Maxwell, Boltzman, Einstein, and Schrodinger, and perhaps more recently to Fitts and Hicks. One aim of our research is to have a more “unified theory” particularly between control, communications, and computing that involves both layers and levels. This involves exploiting diversity, rather than avoiding it as in more primitive theories.
What virtual memories do is exploit the diversity in the memory hierarchy to create a diversity-enable sweet spot (DeSS) that is fast, large, and cheap despite having no such parts, with the fragility that certain rare access sequences could be very slow. Our view is that the most important function of complex architectures is to create DeSS where simpler uses of components cannot. Laws are highly domain specific, but the architectures to cope with them are remarkably universal in their use of layers, levels, and DeSS.
Our cells, organs, bodies and brains also illustrate layered architectures in myriad ways. Our central nervous system (CNS) has the brain/brainstem/spine as its minimal layers, with neurons and other cells as the main lower level. What the brain/stem/spine and apps/OS/HW have in common is a DeSS for flexibility and speed from highly constrained parts. Maximum speed requires special purpose hardware in our spines or computers, but the greatest flexibility is in our apps and brains layer, where apps, memes, and ideas are highly swappable via shared languages. Most hidden but essential are the OS and brainstem to virtualize our fast hardware. Experts estimate that conscious thought is limited to 100 bits/sec bandwidth, whereas the optic nerve alone runs at 10Mbits/sec. This and most data are not discarded but used unconsciously and automatically to drive sensorimotor control, and direct the attention of that tiny and precious conscious thought.
Our cells have a familiar DNA/RNA/protein layering that also emphasizes the molecules that implement the layers, with a universally shared genome by incredibly diverse cell types which are organized to create remarkable DeSS. A major difference is that cells and bodies have their own internal supply chains to make new proteins and cells, whereas our computer hardware is produced “out of band.” A similarity is despite the importance of “code” in DNA and software, hardware and proteins are necessary for the code to function. And some terminally differentiated cells in our blood, eyes, and skin jettison their DNA, RNA, and associated molecular machinery. As in our technologies, specialized hardware solutions provide superior performance but with a loss of flexibility.
Apps, genes, and memes are easily mutated and even swapped, enabling software and genetic engineering as well as education and culture, but a strikingly common fragility is that pathogens can also “swap in” their code. This infectious hijacking leaves the architecture largely intact but switches the function to benefit the pathogen. Bacteria use gene swapping (called “horizontal gene transfer”) to rapidly share antibiotic resistance genes that hijack other elements of the architecture (e.g. G-protein coupled signal transduction protocols). The eukaryotic malaria parasite has a complex lifestyle involving infectious hijacking of mosquitos and mammals. Experts claim that nearly half of the 100G humans who have ever lived were killed by malaria, with modern treatments reducing that rate. However, other so-called “superbugs” are evolving with swappable code that defeats available medical treatments.
Perhaps most ominous for humanity is our affinity for sharable “memes” containing ideas that are attractive and highly infectious, but false and dangerous. Experts argue the very falsehood of memes can sometimes facilitate their transmission, as their costly deleterious effects on their hosts make them honest signals of group membership. This is aggravated by the unpleasant fact that accepting truths often requires short term sacrifices by individuals. Combined with advanced technologies enabling powerful communication, computing, and weaponry, “bad memes” may make malaria’s history seem relatively tame. Ironically, science is not immune to such infectious hijacking, as the enormous popularity of, say, scale-free and related complexity/network science “memes” illustrates. The scale-free meme seems to have become both disdained and chronic in biology and the social sciences, with a persistent reservoir in physics.
Case studies illustrating layered bowtie and hourglass architectures
Our previous work has had success in tackling layering, architecture, and evolvability in several specific cases, but in drastically different scales and contexts. In the internet and its wild evolution, our theory of layering as optimization (LAO) for network utility maximization (NUM) formalized how high layer applications run on diverse hardware virtualized by the universal TCP/IP protocols playing the role of the network OS [1,2,3,4,5]. We also showed how bowtie/hourglass architectures [7,8,9,10] explain the control and evolution of bacterial signal transduction [6], metabolism[8,12], stress response[11], and cell size and composition [14]. We have online videos in addition to the papers below and can be available to anyone who is interested in this background.
1. Doyle et al (2005): The “Robust Yet Fragile” Nature of the Internet, PNAS 2005 102(41): 1497-14502
2. Chiang, Low, Calderbank, Doyle (2007): Layering As Optimization Decomposition: A Mathematical Theory of Network Architecture, Proc of the IEEE 2007: 95(1): 255-312.
3. Willinger, Alderson, Doyle (2009): Mathematics and the internet: A source of enormous confusion and great potential. Notices Amer Math Soc 2009: 56:586-59
4. Matni, Tang, Doyle (2015) A case study in network architecture tradeoffs, ACM Sigcomm Symposium on SDN Research (SOSR), 2015.
5. Matni, Doyle (2016) A Theory of Dynamics, Control and Optimization in Layered Architectures, IEEE American Control Conference, 2016.
6. Yi, Huang, Simon, Doyle: (2000) Robust perfect adaptation in bacterial chemotaxis through integral feedback control. Proc. Natl. Acad. Sci. 2000: Apr 25 97(9): 4649-53.
7. Csete, Doyle (2002): Reverse engineering of biological complexity. Science 2002: 295(5560): 1664-1669.
8. Csete, Doyle (2004), Bow ties, metabolism, and disease, Trends in Biotechnology, Vol 22, Issue 9
9. Doyle, Csete (2011) Architecture, Constraints, and Behavior, PNAS, vol. 108, Sup 3 15624-15630
10. Stelling, Sauer, Szallasi, Doyle III, Doyle: Robustness of cellular functions. Cell 2004: 118 (6) 675-685.
11. El-Samad, Kurata, Doyle, Gross, Khammash, Surviving Heat Shock: Control Strategies for Robustness and Performance, PNAS 2005: 102(8): Feb 22: 2736-2741.
12. Chandra, Buzi, Doyle (2011) Glycolytic oscillations and limits on robust efficiency. Science, Vol 333, pp 187-192.
13. Doyle (2016) Even Noisy Responses Can Be Perfect If Integrated Properly, Cell Systems
14. Kempes, Wang, Amend, Doyle, Hoehler (2016) Evolutionary tradeoffs in cellular composition across diverse bacteria, The ISME Journal, advance online publication 5 April 2016
Most recently, we have new applications in smartgrid technology and more relevant here, in neuroscience. Our previous applications used pre-existing theory, largely developed for aerospace applications that were handcrafted for these complex networked systems. In the last few years we have developed a new theory [15,16,16], called System Level Synthesis (SLS), motivated by biology and neuroscience (and future IOT and smartgrid) that for the first time deals with control (including sensing, communications, computing, and actuation) that is sparse, localized, saturating, delayed, noisy, quantized, and distributed (SLSDNQD) (This is the most exciting theory progress in my >40 years as a researcher.)
15. J. Anderson, J. C. Doyle, S. H. Low, and N. Matni, System Level Synthesis, Annual Reviews in Control, 2019.
16. Y.-S. Wang, N. Matni, and J. C. Doyle, Separable and Localized System Level Synthesis for Large-Scale Systems , IEEE Transactions on Automatic Control, 2018.
17. Y.-S. Wang, N. Matni, and J. C. Doyle, A System Level Approach to Controller Synthesis , IEEE Transactions on Automatic Control, 2019
About CCI Events
With a mission of research, innovation, and workforce development, the Commonwealth Cyber Initiative (CCI) focuses on the intersection of security, autonomous systems, and data. Funded by the Commonwealth of Virginia, CCI is a highly connected statewide network that engages institutions of higher education, industry, government, and nongovernmental and economic development organizations. CCI’s network includes 39 higher education institutions and 320 faculty members as well as more than 20 industry partners. CCI was established in the 2018-20 Virginia budget with an investment of approximately $20 million annually from 2020 and beyond. Follow us on Twitter, LinkedIn, Facebook, Instagram, and YouTube.
Thanks for your interest! Please contact Kendall Beebe at kwbeebe@vt.edu to get involved.
Please email Kendall Beebe at kwbeebe@vt.edu.
CCI posted a recording of the webinar, which you can watch on CCI’s YouTube channel.