CCI Researchers Building Trust in AI
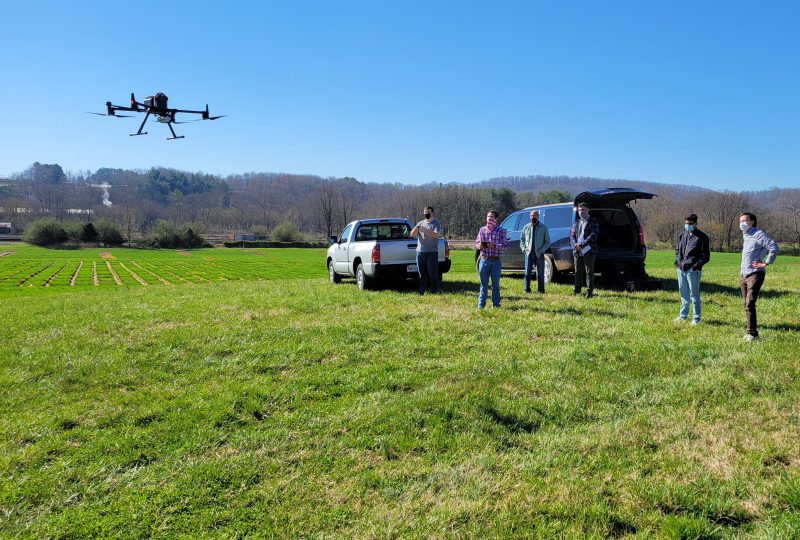
Commonwealth Cyber Initiative (CCI) faculty researchers Laura Freeman and Feras Batarseh are defining what it means to trust artificial intelligence (AI) algorithms in this new and influential field.
AI algorithms can sift through mountains of information, swiftly making connections to respond to changing economic markets, guiding robots in the battlefield, or knowing when an autonomous vehicle should swerve to avoid an accident. But the information is only as good as the trust humans can place in its ability to make these decisions. Fairness, bias, ethics all come into play when building trust, along with simply explaining how the algorithm reaches its conclusions, said Freeman, who’s also the director of the Intelligent Systems Lab at the Ted and Karyn Hume Center for National Security and Technology.
“We’re striving for data-driven decision making that people can trust,” Freeman said. “AI will stay in the realm of making suggestions in our Netflix queue and playing chess games with computers until we can assure it to a level where we trust AI.”
Decisions made by AI also are only as good as the data, noted CCI AI Assurance Testbed Director Abdul Rahman during a Northern Virginia Technology Council event about AI. Poor data leads to poor decisions, he said.
The CCI team will be using the CCI AI Assurance Testbed, which is unique in Virginia, to test data sets for such issues as bias.
This relatively new field is called AI Assurance. Freeman and Batarseh, a CCI research associate professor, have literally written the definition and are writing the book, along with publishing research papers, and working with students.
Students are a vital part of building the workforce pipeline that the field needs. Virginia Tech graduate and undergraduate students are working with the CCI team on the following projects:
- Machine learning model-specific assurance, for models such as genetic algorithms and reinforcement learning
- Cyber-physical systems and national infrastructure security using AI Assurance
- AI Assurance for public policy in agriculture, smart farms, and economics
- AI Assurance modeling for cybersecurity
AI Assurance sub areas, domains, and goals
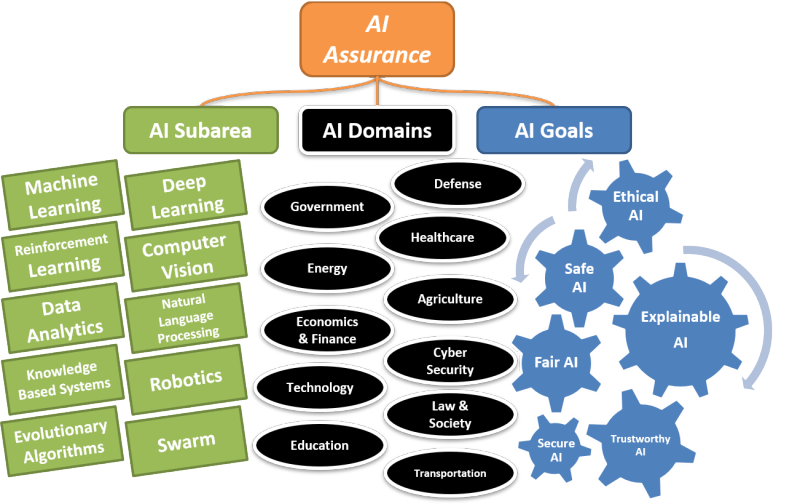
The CCI team is working on AI Assurance for defense, smart farms, critical infrastructure, and public policy where AI can have a transformative impact.
For example, AI and smart farming are helping agriculture become more efficient and precise by monitoring soil, moisture, and weather. AI is also used to help predict the impact of global events that affect prices, consumption, and production, Batarseh said.
In the military, AI algorithms can monitor situations, evaluating for irregular behaviors or activity that could indicate a serious problem such as a coup. “Right now, we have humans staring at screens, but Al models can be used to identify these situations and alert humans,” Freeman said.
AI also can analyze how events can impact public policy and the effects of those policies on society. An ability to connect events to outcomes reliably supports data-driven decision making, she added. But those decision makers need to understand the process of the algorithms and the fidelity of the data that feeds them.
How to measure trust between humans is a field in itself. Add to it, trust between machines and humans and the variables increase.
CCI’s AI Assurance team is creating models that set a goal and create dimensions to measure such as bias, across fields like race, gender, or even simple imbalance in the data that can result in bias across seemingly unlikely variables to trigger biases, Freeman said.
“We go out and collect data and evaluate,” she said. “Then we rinse and repeat.”
CCI’s AI Assurance team members are publishing papers to help build the foundation for AI Assurance. Examples include Test and Evaluation for AI, A Tutorial on the Planning for Experiments, A Survey on Artificial Intelligence Assurance, Graph Neural Networks for Modeling Causality in International Trade.
Defining AI Assurance
AI Assurance is the probability that a system leveraging AI algorithms functions only as intended and is free of vulnerabilities throughout the life cycle of the system. High levels of assurance stem from all the planned and systematic activities applied at all stages of the AI engineering lifecycle ensuring that any intelligent system is producing outcomes that are valid, verified, data-driven, trustworthy and explainable to a layman, ethical in the context of its deployment, unbiased in its learning, and fair to its users.
Laura Freeman and Feras Batarseh