CCI Funds 22 Cybersecurity Research Collaboration Grants
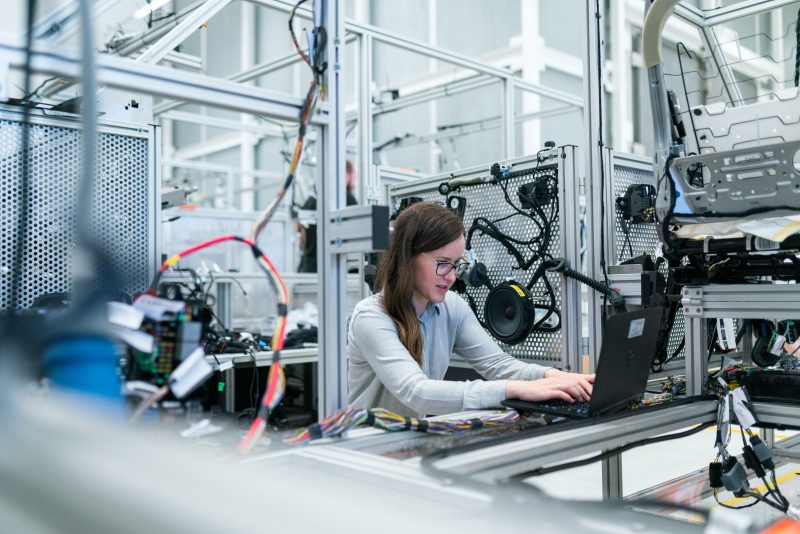
The Commonwealth Cyber Initiative has funded 22 research proposals for a total of $4 million, the initiative announced Dec. 18, 2020.
“These research proposals went through a highly competitive process to achieve funding and show the depth of the research capability within the Commonwealth,” said Luiz DaSilva, CCI executive director. “The 22 collaborative projects will help further the expansion of autonomous systems, AI, 5G applications, and new approaches to security.”
Each of these projects represents a collaboration between faculty members at two or more universities in Virginia, including George Mason University (GMU), James Madison University (JMU), Old Dominion University (ODU), University of Virginia (UVA), Virginia Commonwealth University (VCU), Virginia Tech (VT), and William & Mary. MITRE Corp. and the Global Center for Automotive Performance Simulation (GCAPS), an affiliated company of Virginia Tech, also are participating.
CCI has a Hub and Node structure––the CCI Hub coordinates the entire network and the four CCI Nodes focus on specific regions in Virginia.
Organized by node, the funded projects are:
Project Title: 5GEM: 5G MEC-Enhanced C-V2X for Intersection Management
Principal Investigator: Duminda Wijesekera, professor, computer science, George Mason University
Co-Principal Investigators: Jeffrey Reed, CCI chief technology officer, Willis G. Worcester Professor of electrical and computer engineering, Virginia Tech; Vijay Shah, research assistant professor, electrical and computer engineering, Virginia Tech; Steve Kan, director, CRASH Center, George Mason University; Bo Yu, post-doctoral associate, computer science, George Mason University
- Abstract: The primary goal of this proposal is to design, prototype and perform in-field experimental run of a 5G multi-access Edge Computing (MEC) server for Intersection management and the effect of age of information has on that design. Most CV2X and intersection management address motorized vehicles, and pedestrians at zebra crossings. But they do not consider other emerging modes of urban transportation such as bicycles and motorized scooters for short distance rides. One of the distinguishing components of our proposal is to develop an MEC for intersection management that includes motorized vehicles, pedestrian, bicycles and motorized scooters. Our usage scenario will receive the individual state information from all actors approaching an intersection, including their intended destination across the intersection and cellular broadcasting the most usable information for these three types of actors. To be useful, we will provide algorithms that can filter only the contextually relevant information to directly support their described destinations that minimize the delays at the intersections. We will develop in-lab, in simulation and in-field experiments that would be carried throughout the process to validate our designs, protocols and algorithms and test their viability, cybersecurity and performance in the real-world. We will collaborate with the Arlington County Department of Transportation in selecting the intersections of concern, and carry out the in-field testing at the Rosslyn headquarters.
Project Title: Collective and Collaborative Defense for Virginia Regional Cyber Ecosystems
Principal Investigator: J.P. Auffret, associate director, Center for Assurance Research and Engineering (CARE), George Mason University.
Co-Principal Investigators: Kevin Heaslip, associate professor, civil engineering, Virginia Tech; Abdul Rahman, CCI AI Testbed Director; Jamil Jaffer, executive director, National Security Institute, George Mason University; Karl Darin, VP, operations, ConnectedDMV; George Thomas, VP, innovation and strategic initiatives, ConnectedDMV
- Abstract: The Commonwealth of Virginia has enhanced its cybersecurity posture over the last five years but scaling challenges remain given Virginia’s varied regional critical infrastructure, supply chains and cyber ecosystems. In addition, the work from home environment resulting from the global COVID‐19 pandemic and increased cyber threat surface area due to the adoption of IoT devices and the rollout of 5G infrastructure are furthering the scaling challenge. The project brings together Northern Virginia and Southwest Virginia CCI nodes with the George Mason Center for Assurance Research & Engineering, Volgenau School of Engineering, National Security Institute, Antonin Scalia Law School, Virginia Tech Hume Center for National Security and Technology, the CCI AI Testbed, and Connected DMV. The project proposes adapting the fusion center concept to regional collective and collaborative defense with Regional Cyber Centers (RCCs) by developing innovative strategic, business, legal, policy, and operational constructs that are tailorable for a region’s cyber ecosystem. The project will tailor the RCC model to the Greater Washington Metropolitan Area (DMV) and Blacksburg/New River Valley Planning District and provide a foundation for standing up the RCCs in 2022. The CCI AI Test Bed will develop and demonstrate capability to assist RCCs in scaling including the application of AI‐ML to network sensor feeds for anomaly detection and threat identification; leveraging the Test Bed for both ingest of relevant data and usage of models for prediction, correlation, and analysis of threats, their severity, their impact; and enriching ingested data with other local data to establish common operating pictures.
Project Title: Enhancing 5G Wireless Network Security with Reconfigurable Intelligent Surfaces
Principal Investigator: Kai Zeng, associate professor, Department of Electrical and Computer Engineering, George Mason University
Co-Principal Investigators: Brian Mark, professor, electrical and computer engineering, GMU; Lingjia Liu, associate professor, electrical and computer engineering, Virginia Tech; Changqing Luo, assistant professor, computer science, Virginia Commonwealth University
- Abstract: The concept of reconfigurable intelligent surface (RIS) has recently gained much research attention for beyond 5G technology applications. RISs are man-made surfaces of electromagnetic material that are electronically controlled with integrated electronics and have unique wireless communication capabilities. Although key benefits of RISs in beyond 5G applications have been demonstrated for enhanced capacity, spectral efficiency, and higher rates, its benefits for enhancing 5G wireless network security have not been well understood or explored. This project aims to build theoretical foundations and develop novel techniques for RIS-enhanced 5G wireless security that fully harvest the degrees of freedom provided by RISs. It consists of four research thrusts: 1) to develop a reinforcement learning (RL)-agent assisted physical layer key generation scheme for RIS-enhanced 5G wireless communications; 2) to investigate the use of LPD/LPI waveforms in conjunction with RIS to improve the security of 5G communications and increase spectrum utilization as a cognitive underlay; 3) to design efficient RIS-transmitter/receiver channel state information (CSI) estimation methods to support secure communication; 4) to build an RIS mmWave 5G testbed to evaluate and validate the proposed ideas. The proposed research is well aligned with the strategic focus areas at the CCI NoVa Node, including Strategic Focus Area 1.A.ii: Cyber Research for the National Defense and Initiative 1.B.i: 5G-enabled Test Beds. Part of the research results will be further developed as training and course materials. The developed testbed will also be made available to the CCI partners for training, teaching, and research activities.
Project Title: RSA-DC: Building Robust and Self-Adaptive Defense Capability in Cyber Systems
Principal Investigator: Songqing Chen, professor, computer science, George Mason University
Co-principal Investigator: Qun Li, professor, computer science, College of William and Mary
- Abstract: Most of our services today, ranging from the entertainment to the military, rely on various cyberinfrastructures. As such, attacks targeting cyberinfrastructures, and the corresponding damage and loss caused, keep increasing. While continuous efforts are being made to defend against such attacks, the arms race between attackers and defenders is getting more and more intensive. Most defenses are still mostly static. The efficiency and effectiveness of such defense systems are often heavily relying on the operator and domain experts and their knowledge and experience. Therefore, such systems often cannot keep pace with the attackers. This project proposes to build a RSA-DC, a framework that builds robust and self-adaptive defense capability for existing cyber systems. RSA-DC is built on top of the latest network technology and machine learning and AI techniques. To achieve this goal, the researchers plan to first leverage the software defined networking (SDN) and programmable data planes to achieve low overhead network event collector. Second, to minimize the delay and errors introduced by manual operations of operators, they propose to build a bias learning based dual reinforcement learning model to automate the operation of the systems based on the collected events. Such a model can self-adapt to the monitored events by guiding the operations of the defense system automatically. The researchers plan to build a prototype on cloud data center networks. Note RSA-DC does not exclude experts’ experience and domain knowledge. Instead, it aims to utilize such important knowledge more effectively in an automatic and self-adaptive manner.
Project Title: Spatiotemporal G-code Modeling for Additive Manufacturing Security
Principal Investigator: Rob Prins, engineering professor, James Madison University
Co-Principal Investigator: Irfan Ahmed, assistant professor, computer science, Virginia Commonwealth University
- Abstract: Smart manufacturing includes interpretation of digitally communicated designs into code intended for execution on connected devices such as those associated with additive manufacturing. Simulated cyberattacks on 3-D printers have demonstrated that mechanical properties of a part can be maliciously modified without causing defects that are apparent to the typical inspection process. Such concealed defects are of concern because they may result in parts failing after they are integrated into a larger system and deployed. Failure in this mode jeopardizes the entire system. Researchers have investigated ways to detect cyberattacks on manufacturing code (G-code) including methods that predict expected 3-D printer behavior based on known good code and compare these expectations to observed reality during the build process. The proposed research includes two thrusts. The first thrust will focus on development of several cyberattack simulations applicable to 3-D printers (including generation of internal voids and manipulation of process temperatures). The effect of these different attacks on mechanical properties will be observed through testing. The second thrust will focus on G-code modeling to predict printer behavior; G-code models will be compared to actual printer behavior using an integrity-checking framework. Actual printer behavior will be monitored via a novel technique that is more direct than methods currently described in the literature.
Project Title: User-Centric Privacy Controls for Smart Home Devices
Principal Investigator: Vivian Motti, assistant professor, information sciences and technology, George Mason University
Co-Principal Investigator: Carol Fung, associate professor, engineering, Virginia Commonwealth University
- Abstract: Smart home technologies include an ecosystem of devices aimed to provide convenient services for end users. Smart home devices (SHDs) vary on their form factor and purposes, serving as personal assistants for communication, entertainment, lighting, surveillance, among other services. SHDs are well-suited to automate services and manual activities, however due to their continued data collection, access to personal information from end users, and communication with third-party services through the web, these devices also threaten users’ privacy. Privacy concerns for smart homes are well-acknowledged in the scientific literature and popular media, however addressing privacy risks for SHDs remains an open challenge. To advance the research and development of user-centric controls for smart home devices, this project employs user-centric design. The project aims to systematically characterize privacy concerns from end users’ perspectives, formalizing privacy risks in a threat model for smart home devices. Also, the research team will define, develop and evaluate a privacy-enhancing framework for smart homes. The project contributions are three-fold: (i) a threat model of privacy risks for smart home devices; (ii) graphic user interfaces for privacy controls; and (iii) a catalog of privacy-preserving policies and design patterns. The project is in line with CCI goals investigating the impact of human behavior on cybersecurity focusing on privacy for smart home devices.
Project Title: A Systematic Evaluation of Smart City Security and Privacy
Principal Investigator: Adwait Nadkarni, assistant professor of computer science and director of the Secure Platforms Lab (SPL), The College of William & Mary.
Co-Principal Investigator: Yuan Tian, assistant professor, computer science, University of Virginia
- Abstract: Fueled by lessons learned from small-scale deployments (e.g., smart homes), we are beginning to see efforts at realizing the smart city vision—futuristic cities with various levels of autonomy and cyber-physical control over traditionally manual processes. However, the security and privacy implications of such large-scale deployments are understudied, with the primary challenge being their scale and complexity, caused by the deployment of devices and services with varied technology stacks, and for heterogeneous use cases, which is far beyond relatively small smart home deployments. This proposal seeks to bridge this gap by developing systematic methods to investigate the security and privacy of smart public spaces, i.e., larger deployments of IoT devices (e.g., in office buildings/streets) that constitute smart cities. We propose an empirical approach for evaluating smart public space deployments by formulating two research goals: (1) evaluating the security of network communication, and (2) analyzing the privacy implications of centralized management, particularly in terms of what administrators can observe, and how users perceive such observations. Our scalable research methodology systematically leverages well-founded techniques such as static and dynamic analysis, formal modeling, statistical language modeling, natural language processing, and user-studies. While our approach is general, we aim to evaluate it using the UVA Living Link Lab, a large-scale smart public space testbed. If successful, this proposal this proposal will develop a methodological understanding of the security and privacy challenges in smart city adoption, and an empirical foundation for future research on secure IoT communication as well as usable and privacy-preserving interfaces.
Project Title: Backdoor Detection and Mitigation in Deep Neural Networks
Principal Investigator: Hongyi Wu, Batten Chair of Cybersecurity and the director of the Center for Cybersecurity Education and Research, Old Dominion University
Co-Principal Investigators: Jin-Hee Cho, associate professor, computer science, Virginia Tech; Leigh Armistead, president, Peregrine Technical Solutions; Dave Wolfe, vice president, Peregrine Technical Solutions; Tom Murphy, Peregrine Technical Solutions; Chunsheng Xin, professor, electrical and computer engineering, ODU; Jiang Li, professor, electrical and computer engineering, ODU; Rui Ning, research assistant professor, cybersecurity, ODU
- Abstract: Artificial Intelligence (AI) is becoming a critical part of modern warfare, with its game-changing capability for handling large volumes of data and making collaborative complex decisions in support of self-control, self-regulation, and self-actuation of combat systems. While it is being adopted as an effective tool for automation where, relative to human intelligence, AI is faster, more agile, or low-cost, it is becoming an increasingly attractive target, opening vulnerabilities and enabling new forms of cyberattacks. In particular, a wide range of deep learning algorithms are vulnerable to polluted data, adversarial inputs, mimicry attacks, evasion attacks, and poisoning attacks. The resulting deep learning models are embedded with neural backdoors, that can cause catastrophic failure. The overarching goals of this proposed project include: (1) investigating existing and emerging neural backdoor attacks, (2) exploring and implementing effective backdoor detection schemes, and (3) once detected, developing and testing efficient backdoor mitigation techniques. Researchers from two CCI nodes (Coastal and Southwest) will work with defense industry and Navy/Army collaborators to develop the proposed backdoor detection and mitigation system, contributing significantly to the protection of future intelligent combat systems. The project will also develop new training modules in the context of secure and safe AI and offer them via workshops and bootcamps, aiming to prepare students and practitioners with advanced secure AI skills to succeed in cutting-edge research and industrial projects. Overall, the proposed work will lead to enabling technologies to secure AI systems, accelerating their development and widening their adoption in various application domains.
Project Title: DeepPOSE: Securing Transportation systems from GPS Spoofing Attacks
Principal Investigator: Chunsheng Xin, professor in the Center for Cybersecurity Education and Research, Old Dominion University
Co-Principal Investigators: Yanxiao Zhao, associate professor, electrical and computer engineering, Virginia Commonwealth University; Kevin Kefauver, technical director, Global Center for Automotive Performance Simulation; Danella Zhao, associate professor, computer science, ODU
- Abstract: Today the Global Positioning System (GPS) service is widely used in our daily lives. Smartphones, wearable devices, aircrafts, unmanned aerial vehicles (UAVs), self-driving cars or autonomous vehicles all rely on GPS to benefit from location based services, e.g., navigation, truck/vehicle monitoring, reporting self location in emergency or for rescue, searching nearby gas stations, restaurants, hotels, etc. While GPS becomes an indispensable element in our daily lives, it is rather vulnerable to GPS spoofing attacks even by low-cost hardware, resulting in potentially life-threatening impact. While there has been a long history of studying GPS spoofing, previous solutions to detect GPS spoofing were either applicable to limited scenarios only, or not effective to smart attackers. Furthermore, new challenges, such as varying attack surfaces and lack of mitigation techniques, make the problem even more challenging today. Capitalizing on a strong academia-industry partnership, a team of faculty from ODU and VCU and engineers from GCAPS VTT LLC proposes a holistic framework DeepPOSE to utilize multimodal sensor data to detect and mitigate GPS spoofing attacks to transportation systems, using Deep Learning technologies and emerging wireless communication techniques. The developed system will be prototyped and integrated into the product of GCAPS, a leading vehicle simulation framework, and results in potentially large economic impact on the Commonwealth of Virginia.
Project Title: Secure and Privacy Preserving 5G Network for Connected Vehicles
Principal Investigator: Sachin Shetty, associate director, Virginia Modeling, Analysis, and Simulation Center, Old Dominion University
Co-Principal Investigators: Cong Shen, assistant professor, electrical and computer engineering, University of Virginia; Duminda Wijesekera, professor, computer science, George Mason University; Kai Zeng, associate professor, electrical and computer engineering, GMU; Tan Le, research assistant professor, artificial intelligence, ODU; Jeffrey Reed, CCI's chief technology officer and the Willis G. Worcester professor of electrical and computer engineering, Virginia Tech
- Abstract: The integration of 5G and IoT technologies will result in connected vehicular networks that can profoundly change logistics management in the maritime and transportation industry. However, deployment of 5G empowered vehicular networks will also introduce attack surfaces targeting 5G-IoT ecosystem. This proposal aims to develop a secure and privacy-preserving 5G network capability in connected vehicular networks that can provide trusted information to maritime and transportation domains while protecting networked systems from being abused by malicious actors. Although there are several research efforts in 5G security, several research challenges that need to address to realize an integrated secure and privacy-preserving 5G-enabled vehicular network. In this proposal, we address specific challenges in securing 5G-enabled vehicular networks due to dynamic mobility, unknown and changing deployment environment, open wireless medium and limitations of centralized security infrastructure. Our specific differentiators include: (a) To maintain the effectiveness in changing deployment environment, federated learning can be automatically and continuously updated to fit the needs of specific deployment with no additional cost; (b) We provide an integrated security and privacy enhancing capability that will ensure maximum participation from end users; (c) Our Blockchain assisted distributed trust management employs advanced artificial intelligence approaches for data- and entity-centric trust models to ensure security of messages and vehicle trust; (d) Federated learning is integrated in our delay-aware CAN bus intrusion detection to build a robust and resilient distributed detection; (e) Finally, our solutions are deployed in resource constrained devices, such as, software defined radios, that will reduce cost while maintaining security effectiveness.
Project Title: Virginia State Investments in Port of Virginia: A Simulation-based Framework for Identifying, Assessing, and Mitigating Systemic Cybersecurity at the Operational Technology Layer
Principal Investigator: Rafael Diaz, research associate professor, supply chain digitalization, Old Dominion University
Co-Principal Investigator: Haiying Shen, associate professor, systems & information engineering, University of Virginia
- Abstract: The modernization of terminal operations and facilities and the increased use of intermodal transportation at the Port of Virginia is illustrated in their Capital Investment Plans and the 2065 Master Plan. Significant investments are focused on electrification and promoting the use of alternative intermodal transportation. This transformation entails a shift towards the extensive use of operational technology (OT) and digitalization. The evolution toward cyber-physical systems (CPSs) increases the complexity of operations as devices extensively require reliable communication, coordination, and control systems. The emergence of CPSs and extensive use of OT inexorably increases operational complexity and exposure to cyberattacks. Regrettably, many OT systems are not designed against unprotected intrusion, and collectively, may represent a substantial source of supply chain disruption. These industrial OT systems are characterized by the presence of hierarchies and hyper-vulnerabilities at different operational levels. Cybersecurity protection of these systems requires a systemic approach to evaluate their vulnerability, cyberthreats, and countermeasures. However, current evaluating technologies are applied mainly to IT systems and commonly lack systemic perspectives that consider overlapping risks and tiered hierarchies at the OT layer. Particularly, as an extensive volume of vehicle movements occur at different stages of arrival, transit, and departure of cargo, false information attack of vehicle communication is of preponderant attention. With a view to overcoming these limitations, we propose to develop a simulation-based framework to gain a systemic cybersecurity perspective of the diverse and interconnected OT systems that define the CPS ecosystem of the Port of Virginia with a focus on vehicle communications.
Project Title: C3-5GPG: Cybersecure Communications and Control for 5G-enabled Power Grid
Principal Investigator: Ali Mehrizi-Sani, associate professor, electrical and computer engineering, Virginia Tech
Co-Principal Investigators: Duminda Wijesekera, professor, computer science, George Mason University; Vijay Shah, research assistant professor, electrical and computer engineering, VT
- Abstract: The overarching goal of this research proposal is to create a cybersecure distributed control, protection, and monitoring infrastructure for distributed energy resources (DER) within a microgrid power system. We capitalize on the URLLC feature of 5G communication and further evaluate our proposed algorithms via both simulation studies and 5G and power grid testbed experiments. The salient aspects of this architecture are its cybersecurity and distributed nature, which coupled with the promise of 5G communications are expected to enable a paradigm shift in power system control. The current power system practice uses proprietary communication assets to handle high-reliability applications at the transmission and generation levels. However, this legacy approach invariably limits future expansion of the system, requires specialized personnel training, and lacks interoperability. Simultaneously, for long, the power community has avoided reliance on commercial communication technologies at the distribution level because of cost of equipment, security/privacy concerns, and lack of performance. 5G communication infrastructure, however, has the promise of enabling such applications via its URLLC capabilities. In this project, we investigate the impact of practical 5G communication, considering both its promise and limitations, on the power system. We then optimally design cybersecure low-latency communications and control for 5G-enabled power grid. Our specific objectives are (i) designing coordinated control of DERs in a microgrid, (ii) optimizing 5G communications for the microgrid application, (iii) enhancing cybersecurity of 5G based communication, and (iv) evaluation via experiments and testbed demonstration.
Project Title: Enhancing the Privacy and Reliability of Massive-scale Bluetooth Low Energy Contact Tracing
Principal Investigator: Daphne Yao, associate professor, computer science, Virginia Tech
Co-Principal Investigators: Carol Fung, associate professor, engineering, Virginia Commonwealth University; Tijay Chung, assistant professor, computer science, VT
- Abstract: Numerous studies have shown the importance of early detection and quarantine in preventing the spread of the deadly COVID virus. However, technology-based contact tracing is grossly undervalued and underutilized. We will examine and harden the security, privacy, and reliability of contact tracing mobile apps, with a focus on Virginia’s COVIDWISE contact tracing app. COVIDWISE is based on Google and Apple’s Exposure Notification System, which utilizes Bluetooth low energy (BLE) technology to broadcast Bluetooth beacons from one device to another when mobile users come in close proximity. Our rigorous security and privacy analysis will have two focuses: (i) to ensure that the library code (from Google and Apple) and the application code (from various government and health organizations) properly protect user privacy and (ii) to harden the design and implementation, if necessary. Our preliminary work has identified beacon-related deficiencies on mobile devices. We will conduct comprehensive static and dynamic runtime program analyses and tests to inspect all aspects of the operations, including data and control flows, data storage, cryptographic key generation, and network communications. Equally important, we will explain and democratize the knowledge of BLE-based contact tracing to the general public and advocate for the wide use of contact tracing apps within the Commonwealth. The team has deep expertise in mobile and network security and is strongly determined to contribute to the well-being of Commonwealth residents. Our project will help address the early infection detection challenge in this unprecedented global pandemic and contribute to the Commonwealth’s economic recovery.
Project Title: Evaluation of Lattice-Based Candidates in the NIST Post-Quantum Cryptography Standardization Process in Terms of Security and Performance in Hardware
Principal Investigator: Travis Morrison, assistant professor, mathematics, Virginia Tech
Co-Principal Investigator: Kris Gag, professor, cryptographic engineering, George Mason University
- Abstract: A large-scale quantum computer will break all currently deployed public-key cryptosystems. To prepare for the coming “post-quantum” world, The National Institute of Standards and Technology (NIST) is running a process to standardize one or more public-key cryptosystems which are secure even against an adversary with a quantum computer. Round 3 of this process officially began October 23, 2020 and will last 12-18 months, perfectly overlapping with the period of activity of this call for proposals. The PIs will implement in hardware and benchmark lattice-based cryptographic algorithms which are under consideration for standardization. In previous standardization efforts led by NIST, hardware benchmarking proved to be a critical tool for selecting finalists. Implementing these algorithms in hardware requires domain expertise in both engineering and computational number theory due to the complex mathematical algorithms these schemes are based on. The PIs will analyze the security of these implementations by estimating concrete costs of attacking the scheme to an adversary equipped with a quantum computer. Understanding concrete costs (versus mere asymptotic costs) is critical to building confidence in the security of these cryptosystems. The PIs will also analyze the resilience of these protocols against side-channel attacks.
Project Title: O-RAN Compliant User Driven RAN Resource Management System for Next-generation Mobile and C-V2X Communications
Principal Investigator: Vijay Shah, research assistant professor, electrical and computer engineering, Virginia Tech
Co-Principal Investigators: Bo Yu, post-doctoral associate, computer science, George Mason University; Ying Wang, CCI 5G Testbed radio frequency engineer
- Abstract: The future generation mobile network is expected to support various types of services such as eMBB (enhanced Mobile Broadband), mMTC (massive Machine Type Communications), and URLLC (Ultra-Reliable and Low Latency Communications) and beyond, while at the same time fulfilling different QoS/QoE requirements. These requirements will be determined between the network operator and end-users (human users, autonomous vehicles etc.) with specifications of key performance indicators (KPIs), such as throughput, latency, connectivity, etc. As a service-based architecture, network slicing enables a diverse range of services to be accommodated in the same physical radio access network (RAN). To satisfy service requirements, the key is the placement of distributed RAN resources (e.g, spectrum, computation, memory resources etc.) that support dynamic customization of each slice. In this proposal, we envision to architect an O-RAN complaint user-driven RAN resource management (RRM) system that dynamically predicts network progression and conducts network design (i.e., RAN resource management) strategies and auto-deployment within the network periodically through temporal data mining of the user traffic and mobility behaviors, with a focus on two usecases: human users in 5G network and vehicles in C-V2X scenarios. Our specific tasks are: (i) prototying intelligent user-driven RRM system, (ii) Adapting user-driven RRM system to C-V2X, (iii) ensuring O-RAN compliance design and testing of user driven RRM system, and finally, (iv) Integration and evaluation of user driven RRM system using CCI 5G testbed.
Project Title: Sensor Degradation Detection Algorithm for Automated Driving Systems
Principal Investigator: Michelle Chaka, program director, Virginia Tech Transportation Institute
Co-Principal Investigators: Chunsheng Xin, professor, Center for Cybersecurity Education and Research, electrical and computer engineering, Old Dominion University; Kevin Kefauver, technical director, Global Center for Automotive Performance Simulation
- Abstract: The project will develop a sensor degradation detection algorithm for Automated Driving Systems (ADS). Sources of degraded sensor information include weather, cyberattacks (e.g., direct communication and passive false signage), and sensor malfunction. Incorrect information from a sensor could result in significant safety issues, such as leading the vehicle off the road or causing the vehicle to suddenly stop in the middle of an intersection. From the Virginia Tech Transportation Institute’s (VTTI’s) Naturalistic Driving Database (NDD), 1000 events related to sensor perception will be selected to establish baseline sensor performance. VTTI will then determine performance metrics using these events extracted from the NDD for comparison in simulation. A virtual framework will be used to test degraded sensor states and the response of the vehicle control systems to develop the detection algorithm. The framework will integrate the sensors models, environments, vehicle model, cyberattacks, and algorithm. Old Dominion University will develop the GPS model, which is a localization sensor, and collaborate with the Global Center for Automotive Performance Simulation (GCAPS) to develop the degradation detection algorithm. GCAPS will also create the virtual framework, develop the LiDAR and radar sensor models, and execute the simulations. The sensor degradation detection algorithm will aid ADS vehicles in decision making by identifying degraded sensor performance.
Project Title: SmallSat Cybersecurity and Resiliency
Principal Investigator: Jonathan Black, director, Aerospace and Ocean Systems Laboratory, Hume Center, Virginia Tech
Co-Principal Investigators: Chris Goyne, director, Aerospace Research Laboratory, University of Virginia; Daniel Doyle, research assistant professor, Aerospace and Ocean Systems Lab; Scott Kordella, senior advisor, space systems, MITRE Corp.
- Abstract: The project leverages an existing University Innovation Exchange Commonwealth-wide partnership with MITRE Corp. to address the challenging and increasingly important issue of smallsat cybersecurity. Small satellites are proliferating at an exponential rate, and in the last few years space assets have been designated critical infrastructure by the National Security Space Policy and NIST. NSA-certified Type 1 encryption and other Federal grade cybersecurity measures are impractical for small satellites and experimental manufacturers, and therefore there is a clear need for the proposed working group to develop best practices for smallsat cybersecurity, execute cyber risk assessments, and analyze resiliency on a lab-based evolvable flatsat. This project is therefore Commonwealth-wide from inception, will include the ability for remote access to the flatsat lab capability and integration with CCI testbed(s), and will work with federal and state agencies and companies across the Commonwealth to transition to self-sustainment.
Project Title: Determination of safety limits against cyber threats in neuromodulation devices using machine learning, brain phantoms and neuronal pathways
Principal Investigator: Ravi Hadimani, assistant professor and director of biomagnetics laboratory, Virginia Commonwealth University
Co-Principal Investigators: Jayasimha Atulasimha, Qimonda Professor, mechanical and nuclear engineering, VCU; Hoda Eldardiry, associate professor, computer science, Virginia Tech
- Abstract: There has been a rapid growth in FDA approval of electromagnetic neuromodulation products for diagnosis and treatment of mental disorders. Most of these devices and procedures use an electromagnetic signal to stimulate critical regions of the brain as a treatment procedure and are prone to external malicious attacks either through a network or from multiple high frequency electromagnetic signals originating from multiple sources. Currently, these devices do not have functional safety features that block stimulation parameters that can cause over stimulation and damage the brain regions or stimulate critical brain regions with incorrect dose and duration. We propose to identify the stimulation parameters that fall outside of the safety limits. We also propose to develop device features that will block any excessive stimulation that are higher than the safety limits or that are directed towards brain regions that control critical parts of the human body such as the brain stem. We will use meta-modelling machine learning technique to determine the stimulation parameters that are vulnerable to external attacks.
Project Title: Developing an Open Architecture Testbed and Learning-based Management for Smart Cities (OpenCity)
Principal Investigator: Sherif Abdelwahed, professor, electrical and computer engineering, Virginia Commonwealth University
Co-Principal Investigators: Nasibeh Zohrabi, post-doctoral fellow, electrical and computer engineering, VCU; Ashraf Tantawy, assistant professor, electrical and computer engineering, VCU; Murat Kuzlu, assistant professor, electrical engineering technology, Old Dominion University
- Abstract: In this project, we will develop an open architecture testbed for smart cities, called OpenCity, which consists of data collection and processing units, database management, distributed performance management algorithms, and real-time data visualization. The aim of the proposed smart city testbed is to support various educational and research activities related to smart city development. The testbed will provide a new-real-life platform to allow students to learn about the unique features of smart cities and explore the supporting technologies. In addition, it will facilitate the development, deployment, and validation of new tools and algorithms to support future smart city developments. The proposed testbed will support various ongoing important research directions in smart cities, including smart buildings, urban mobility, smart energy distribution, and water management. In addition, it will be extendable to include other potential applications and components as needed. The developed testbed will be validated by developing and deploying a learning-based management system that focuses on building comfort and resource efficiency. The learning-based management system will integrate model-based predictive control approach and learning techniques to enable real-time management of the complex building system considering various uncertainties including human behavior and environment conditions
Project Title: Open-source, Multi-band, Multi-dimensional Spectrum Access system with Interfaces to Wireless Testbeds and Network Simulation Software
Principal Investigator: Cong Shen, assistant professor, electrical and computer engineering, University of Virginia
Co-Principal Investigators: Carl Dietrich, research associate professor, electrical and computer engineering, Virginia Tech; Nicholas Polys, director of Visual Computing, VT
- Abstract: The main objective of this project is to build an open-source and multi-dimensional spectrum access system (MD-SAS) that covers a wide array of bands that are of practical interest in dynamic spectrum sharing. The sharp focus of this work is on three technical aspects: (1) MD-SAS testbed development and prototyping; (2) Interfacing of the MDSAS with experiment management/ performance measurement software and integration with both hardware testbeds and network simulation and measurement software; and (3) research of a novel hybrid sensing and ARQ design and its realization in the proposed MD-SAS system. These three research activities build on prior work by the PI and co-PI, but are substantially novel contributions that have potential to greatly increase efficiency of radio frequency (RF) spectrum use in shared RF bands. In particular, this project will demonstrate, as a pilot research, that the developed MD-SAS system and its companion network performance evaluation and simulation software can be widely used by researchers in the Commonwealth for their own research efforts in spectrum engineering.
Project Title: Secure, Smart, Point of Care Sensors for Lung Health
Principal Investigator: Rebecca Heise, associate professor and undergraduate program director, biomedical engineering, Virginia Commonwealth University
Co-Principal Investigators: Vamsi K. Yadavalli, associate professor, chemical and life science engineering, VCU; Angela Reynolds, associate professor, mathematics, VCU; Alpha Fowler, William Taliaferro Thompson professor of medicine, VCU; Jennifer Van Mullekom, associate professor, statistics, Virginia Tech; Carl Elks, associate professor, electrical and computer engineering; VCU
- Abstract: Point of Care biosensors are needed for monitoring in diseases affecting the lung, such as sepsis. There is an imperative need to improve data security of these devices as they will be relaying and indicating sensitive information from patient to clinician. Our overall goal is to develop a minimally invasive, secure, smart biosensor that predicts lung health. The goal of this one-year proposal is to advance the technology of smart and secure Point of Care biosensors by focusing on the following objectives: Objective 1: Engineer secure-by-design smart sensor using samples from experimental lung injury models; Objective 2: Develop an experimentally driven computational framework to accurately predict lung injury severity based upon sensor levels. We will utilize our interdisciplinary team to develop secure, smart biosensors that can predict overall lung health by inputting sensor readings into an in silico computational model of lung injury. Coupled with data analytics and data security, the results from this project will broadly advance Virginia’s leadership role in the development of biodevices. The development of secure tools to accurately measure patient biomarkers presents a new opportunity for the development of smart health biodevices that can affect the lives of millions.
Project Title: Smart City Infrastructures for Safeguarding Autonomous Vehicles Against Cyber Attacks
Principal Investigator: Felix Lin, associate professor, Department of Computer Science, University of Virginia
Co-Principal Investigators: Peng Wei, assistant professor, mechanical and aerospace engineering, George Washington University; Lance Sherry, associate professor, systems engineering and operations, George Mason University
- Abstract: This project is to mitigate public safety and security threats imposed by autonomous vehicles as they become victims of emerging cyber attacks; to do so, the project integrates two novel mechanisms: city-scale video analytics for victim detection and multiagent reinforcement planning for collision avoidance. At a high level, this project promotes a co-design of autonomous vehicles and smart-city infrastructures to identify the source of cyber attack and react to the compromised vehicle(s). This project will advance the knowledge of autonomous driving security, foster commercial adoption of autonomous vehicles, and train the Virginia workforce in security and smart transportation. The three principal investigators form a strong team with a unique research idea that fits the Commonwealth Cyber Initiative (CCI)’s priorities. (i) UVA, GMU and GWU are from two different CCI nodes: Northern Virginia (GMU and GWU) and Central Virginia (UVA). (ii) This proposal aims at the intersection of security, autonomous systems, and data, which is the focus of the CCI. (iii) This proposal covers both CCI nodes’ focus areas. Concretely, we address the Northern Virginia node’s research area of cybersecurity in transportation and the Central Virginia node’s research area of cyber physical systems, autonomous systems, IoT and their applications in Smart Cities.