Characterizing Biases in Automated Scam Detection Tools for Social Media to Aid Individuals with Developmental Disabilities
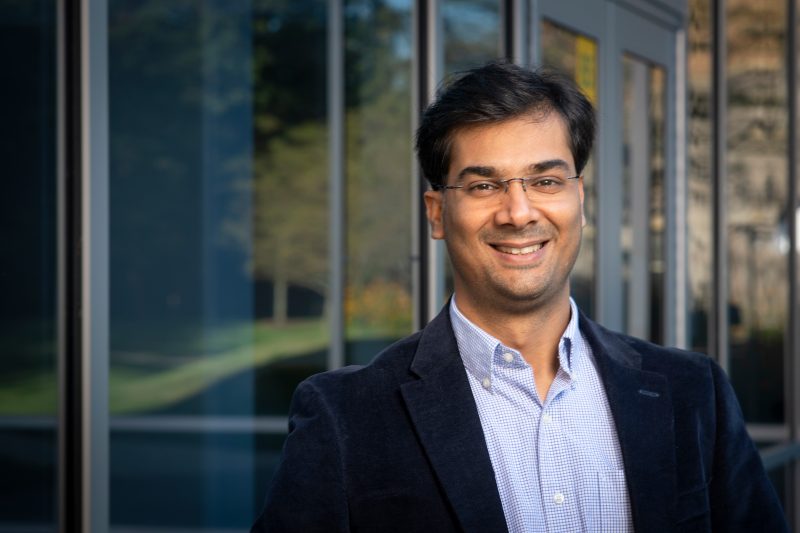
Dr. Hemant Purohit
KEY INTERESTS
Interactive intelligent system design; Human interaction, processing, and management of nontraditional data sources (social media, IoT, etc.); Data mining and social computing; Semantic computing and NLP; Machine learning and human-centered computing
AFFILIATIONS/APPOINTMENTS
Associate Professor, Information Sciences & Technology Department, George Mason University
Director, Humanitarian Informatics Lab, George Mason University
ACADEMIC DEGREES
BS, Communication & Computer Engineering, The LNM Institute of Information Technology
PhD, Computer Science & Engineering, Wright State University
CHARACTERIZIN BIASES IN AUTOMATED SCAM DETECTION TOOLS TO AID INDIVIDUALS WITH DEVELOPMENT DISABILITIES
Cybercrimes have been on the rise in recent years. The number of crimes during times of crisis, ranging from financial fraud to identity theft, that makes use of online social media is increasing annually. For example, when Hurricane Florence hit the U.S. in 2018, people were scammed through social media posts promoting fake relief donation links such as hurricaneflorencerelieffund-dot-com. Throughout the ongoing COVID-19 pandemic, the U.S. National Center for Disaster Fraud has been continually issuing warnings about similar fraud incidents. Individuals with developmental disabilities (e.g., autism, ADHD) are especially vulnerable to such scams, given that they require dedicated types of assistance to help protect them by identifying and avoiding such threats. Researchers in cybersecurity and social computing have developed tools with automated methods to detect scams on social media using natural language processing (NLP) techniques based on machine learning (ML) algorithms. However, the design of these methods relies on a one-size-fits-all approach geared towards protecting the most generic users. The effectiveness of existing methods for scam detection for minority user subpopulations, like individuals with developmental disabilities, is underexplored in the literature. In particular, data labeling as well as features used by ML models to classify scams in social media posts do not consider the behavior of how minority users with developmental disabilities perceive and respond to scam attempts. This is especially concerning given the practice of extensively using automated feature engineering and pre-trained language models for feature representation to achieve state-of-the-art performance in various NLP tasks. Thus, feature representations encoded in models used for NLP techniques for scam detection can often inadvertently perpetuate undesirable biases from the labeled data on which they are trained. The false negatives resulting from such scam detection methods can have serious consequences if employed in assistive tools for individuals with developmental disabilities. This project lays the groundwork for developing inclusive assistive technology for cybersecurity by investigating the way in which the attention of individuals with developmental disabilities differs from generic users when perceiving and responding to scam content on social media during online browsing, including a novel design guideline of data labeling scheme to develop inclusive scam detection methods for social media.