Threat Hunting System Enhancement by Generative AI and LLMs
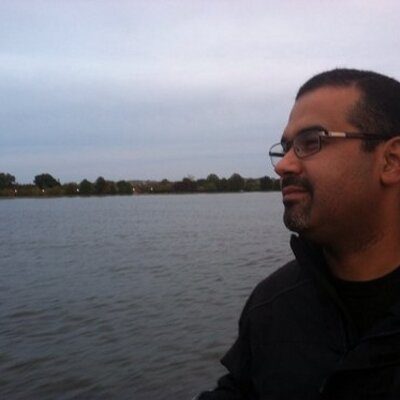
Dr. Mohamed Gebril
KEY INTERESTS
Threat modeling; Cyber-Physical Systems; UAV security
AFFILIATIONS/APPOINTMENTS
Associate Professor, Department of Cyber Security Engineering, George Mason University
ACADEMIC DEGREES
PhD, Electrical Engineering, North Carolina A&T State University
THREAT HUNTING SYSTEM ENHANCEMENT BY GENERATIVE AI
Network security continues to evolve to adapt to the emergence of new adversarial threats, such as prompt injection attached. Further, Artificial Intelligence (AI) tools and generative AI can enhance and automate defense measures. As a result, with the use of threat hunting and anomaly detection utilizing AI (THAD-AI) systems that include generative AI capabilities, network traffic can be more secured and system assets can be protected against adversaries.
This research investigates the impact of generative AI and large language models (LLMs)on automated threat-hunting operations, develops a system for life network traffic monitoring, and performs automated incident response on large real-time data containing suspicious network traffic that is generated based on prompt injection attacks. In addition, incident response is performed by creating incident response cases of flagged anomalies and developing an alert system via detection rules generated by LLMs using the developed THAD system.